The Met Dataset|艺术作品识别数据集|计算机视觉数据集
收藏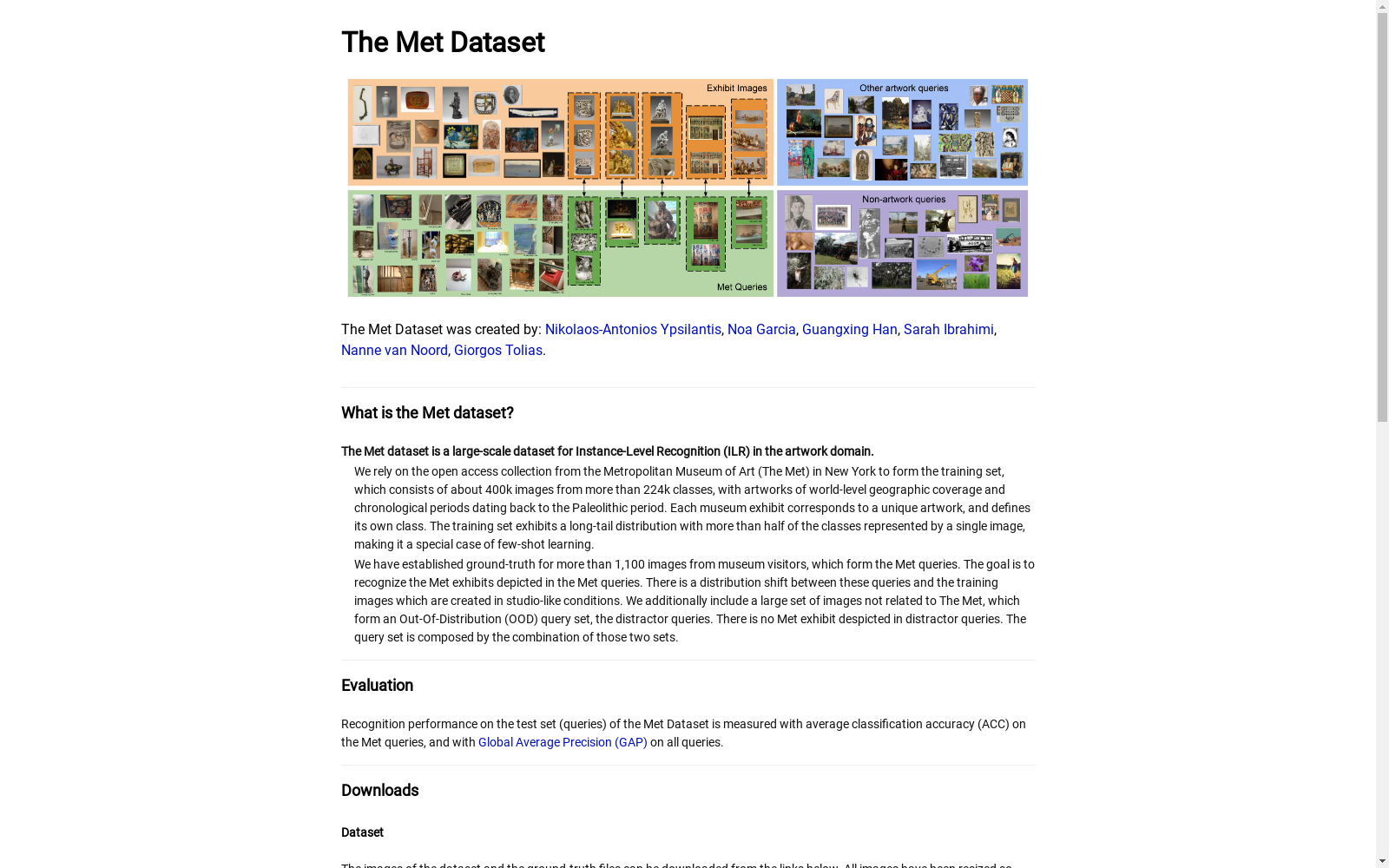
- 1The Met Dataset: Instance-level Recognition for Artworks捷克技术大学电气工程学院 · 2022年
Tropicos
Tropicos是一个全球植物名称数据库,包含超过130万种植物的名称、分类信息、分布数据、图像和参考文献。该数据库由密苏里植物园维护,旨在为植物学家、生态学家和相关领域的研究人员提供全面的植物信息。
www.tropicos.org 收录
URPC系列数据集, S-URPC2019, UDD
URPC系列数据集包括URPC2017至URPC2020DL,主要用于水下目标的检测和分类。S-URPC2019专注于水下环境的特定检测任务。UDD数据集信息未在README中详细描述。
github 收录
AISHELL/AISHELL-1
Aishell是一个开源的中文普通话语音语料库,由北京壳壳科技有限公司发布。数据集包含了来自中国不同口音地区的400人的录音,录音在安静的室内环境中使用高保真麦克风进行,并下采样至16kHz。通过专业的语音标注和严格的质量检查,手动转录的准确率超过95%。该数据集免费供学术使用,旨在为语音识别领域的新研究人员提供适量的数据。
hugging_face 收录
2025年目标检测分割分类数据集大合集
FIRC资源搜索下载工具是一个在windows上使用的工具,可以搜索数据集、whl文件,软件,文档等资源的工具。软件资源会不定时更新欢迎下载使用。 受到文件大小文件限制,请访问:https://aistudio.baidu.com/datasetdetail/325626 直接下载使用,或者去下面云盘也可以: 链接: https://pan.baidu.com/s/1a6r53cXtD9jEaSKdl-TIGQ 提取码: ybay 手势识别检测数据集VOC+YOLO格式2399张6类别 公路隧道积水检测数据集VOC+YOLO格式207张1类别 烟草叶子烟叶病害检测数据集VOC+YOLO格式1555张4类别有增强 陶瓷绝缘子缺陷检测数据集VOC+YOLO格式2908张3类别 绝缘子缺陷检测数据集VOC+YOLO格式795张4类别 瓷砖缺陷检测数据集VOC+YOLO格式888张3类别 瓷砖缺陷检测数据集VOC+YOLO格式2871张7类别 齿轮缺陷检测数据集VOC+YOLO格式7742张3类别 西红柿番茄生长周期检测数据集VOC+YOLO格式4224张5类别 西红柿番茄检测数据集VOC+YOLO格式2320张1类别可用于计数 楼梯上下检测数据集VOC+YOLO格式5462张2类别 电脑屏幕缺陷检测数据集VOC+YOLO格式613张6类别 虾病害检测数据集VOC+YOLO格式923张3类别 捕鱼船检测数据集VOC+YOLO格式2105张1类别 生菜缺乏症缺钙镁氮磷钾检测数据集VOC+YOLO格式2978张6类别 花卉识别分割数据集labelme格式7111张102类别 花卉检测数据集VOC+YOLO格式8402张106类别 水果检测多类水果检测数据集13374张67类别有增强 监控视角跌倒检测数据集VOC+YOLO格式1963张2类别 高质量监控视角跌倒检测数据集VOC+YOLO格式9599张2类别 近距离拍摄沙滩海滩垃圾识别分割数据集labelme格式3432张10类别有增强图片 水下塑料垃圾识别分割数据集labelme格式2703张6类别 垃圾识别分割数据集labelme格式2937张75类别 塑料瓶识别分割数据集labelme格式976张1类别 海洋垃圾分割数据集labelme格式3110张8类别 robotiq机械臂夹爪检测数据集VOC+YOLO格式3026张1类别 人体安全帽反光衣检测数据集VOC+YOLO格式4064张4类别 电动车头盔骑行者检测数据集VOC+YOLO格式2723张3类别 高质量反光衣反光服数据集VOC+YOLO格式5208张4类别 塑料瓶识别检测数据集VOC+YOLO格式3024张1类别 纸质包装盒纸箱包裹损坏缺陷检测数据集VOC+YOLO格式2397张2类别 机场跑道异物检测数据集VOC+YOLO格式33793张31类别 虾新鲜度检测数据集VOC+YOLO格式6313张5类别 鱼类新鲜度检测数据集VOC+YOLO格式3267张2类别 鱼类新鲜度检测数据集VOC+YOLO格式2569张3类别鱼眼判断新鲜度 蜱虫检测数据集VOC+YOLO格式2393张1类别有增强 电力场景输电线路植被被侵占输电线树障检测数据集VOC+YOLO格式2981张2类别 洪涝灾害中人车房建筑检测数据集VOC+YOLO格式9464张3类别 河道违建检测数据集VOC+YOLO格式223张1类别 攀爬行为识别检测数据集VOC+YOLO格式1634张2类别 攀梯行为攀爬行为识别检测数据集VOC+YOLO格式3580张2类别 分心疲劳驾驶检测数据集VOC+YOLO格式22581张12类别 牛脸分割数据集labelme格式1324张1类别有增强图片 牛脸检测数据集VOC+YOLO格式2079张1类别 水下目标检测数据集VOC+YOLO格式1224张7类别 水下垃圾检测数据集VOC+YOLO格式5127张15类别 汽车损伤检测数据集VOC+YOLO格式4000张6类别 汽车损伤分割数据集labelme格式4000张6类别 DIOR遥感目标检测数据集VOC+YOLO格式23463张20类别 道路缺陷裂缝坑洼检测数据集VOC+YOLO格式14569张10类别 exdark检测数据集VOC+YOLO格式7363张12类别 螺栓螺母检测数据集VOC+YOLO格式408张2类别 石榴病害检测数据集VOC+YOLO格式2356张4类别 智慧交通极端恶劣天气晚上雨天雪天雾天道路目标检测数据集VOC+YOLO格式9636张8类别有增强 道路极端天气下目标检测数据集VOC+YOLO格式3191张7类别夜晚雨天2个场景 智慧交通极端恶劣天气晚上雨天雪天雾天道路目标检测数据集VOC+YOLO格式3868张8类别 恶劣天气极端天气道路车辆目标检测数据集VOC+YOLO格式1026张7类别 树上石榴检测数据集VOC+YOLO格式3421张3类别 树上石榴成熟度生长周期检测数据集VOC+YOLO格式5855张5类别 石榴成熟度检测数据集VOC+YOLO格式960张5类别 无人机树木识别及损伤检测数据集VOC+YOLO格式3171张2类别 无人机海洋沙滩海滩垃圾检测数据集VOC+YOLO格式2982张6类别 无人机拍摄烟雾火灾检测数据集VOC+YOLO格式3245张2类别 智慧医疗胃癌检测数据集VOC+YOLO格式487张2类别 智慧医疗消化道疾病检测数据集VOC+YOLO格式3474张3类别 PET透明塑料瓶缺陷检测数据集VOC+YOLO格式366张4类别 樱桃检测数据集VOC+YOLO格式153张1类别 棉花品种分类检测数据集VOC+YOLO格式402张4类别 树上油茶果检测数据集VOC+YOLO格式1677张1类别 X光图像手骨检测数据集VOC+YOLO格式3839张6类别 SIMD数据集遥感地物目标检测数据集VOC+YOLO格式5000张17类别 3D打印缺陷三维打印缺陷检测数据集VOC+YOLO格式5864张3类别 孕妇怀孕周期检测数据集VOC+YOLO格式615张31类别 残疾人员检测数据集VOC+YOLO格式3168张5类别 医学图像分割数据集肺分割数据labelme格式6299张2类别 中空圆柱形缺陷检测数据集VOC+YOLO格式4130张5类别 PLC缺陷检测数据集VOC+YOLO格式1346张3类别 医学图像白血病检测数据集VOC+YOLO格式835张4类别 医学图像白血病分割数据集labelme格式245张5类别 电力场景防震锤缺陷检测数据集VOC+YOLO格式2281张2类别 茄子检测数据集VOC+YOLO格式3129张1类别 RSW螺栓缺陷瑕疵检测数据集VOC+YOLO格式4134张7类别 陶瓷碗破碗缺陷检测数据集VOC+YOLO格式266张4类别 5类URPC2021水下目标检测数据集VOC+YOLO格式7469张5类别 持刀检测数据集VOC+YOLO格式12958张1类别 智慧城市道路防护栏破损缺陷检测数据集VOC+YOLO格式6939张3类别 钢筋计数检测数据集VOC+YOLO格式5610张1类别84万个框 淡水鱼检测数据集VOC+YOLO格式2967张31类别 超全动物检测数据集VOC+YOLO格式27652张80类别 大豆叶子生长周期检测数据集VOC+YOLO格式2400张4类别 生菜生长周期检测数据集VOC+YOLO格式1500张5类别 水稻生长发育周期检测数据集VOC+YOLO格式846张3类别 智慧医疗蛀牙检测数据集VOC+YOLO格式2792张3类别 无人机高空红外数据集VOC+YOLO格式2866张5类别 纽扣电池缺陷分割数据集labelme格式28张2类别 纽扣电池缺陷检测数据集VOC+YOLO格式220张2类别 半导体晶圆缺陷检测数据集VOC+YOLO格式6174张6类别 瓶盖缺陷检测数据集VOC+YOLO格式8524张6类别 瓶盖缺陷检测数据集VOC+YOLO格式565张3类别 建筑工地常用工具检测数据集VOC+YOLO格式2609张49类别 烟火烟雾明火分割数据集labelme格式4065张2类别 金属缺陷检测数据集VOC+YOLO格式3422张3类别 智慧工厂轴承齿轮螺栓螺母检测数据集VOC+YOLO格式2249张4类别 智慧医疗胸肺CT检测数据集VOC+YOLO格式4103张12类别 手语检测数据集VOC+YOLO格式9648张80类别 电力场景绝缘子缺陷分割数据集labelme格式1585张4类别 智慧医疗肝病检测数据集VOC+YOLO格式3964张4类别 水管滴水漏水检测数据集VOC+YOLO格式2708张1类别 举手检测左右手检测数据集VOC+YOLO格式5594张2类别 加油站加油行为规范检测数据集VOC+YOLO格式1136张2类别 自动扶梯人员摔倒掉落识别检测数据集VOC+YOLO格式5375张2类别 水稻叶子病害检测数据集VOC+YOLO格式1059张4类别 水稻叶子病害检测数据集VOC+YOLO格式11290张4类别 水稻叶子病害检测数据集VOC+YOLO格式6138张3类别 牙齿缺陷分割数据集labelme格式2495张4类别 花生病害检测数据集VOC+YOLO格式7245张5类别 莴笋莴苣叶子病害检测数据集VOC+YOLO格式6011张5类别 茄子虫害检测数据集VOC+YOLO格式3911张4类别 物流场所设施检测数据集VOC+YOLO格式4906张4类别 卫星图像烟雾火点检测数据集VOC+YOLO格式8098张2类别 遥感极小目标检测数据集VOC+YOLO格式9814张8类别 三种颜色辣椒检测数据集VOC+YOLO格式10006张3类别有增强 智慧医疗肝脏肿瘤检测数据集VOC+YOLO格式8619张2类别 地质雷达探地雷达检测数据集VOC+YOLO格式874张2类别 农作物叶子病害检测数据集VOC+YOLO格式5694张30类别 农作物叶子病害检测数据集VOC+YOLO格式5169张29类别 水果蔬菜检测数据集VOC+YOLO格式9637张94类别 半导体芯片缺陷检测数据集VOC+YOLO格式5874张5类别 糖尿病视网膜病变检测数据集VOC+YOLO格式1785张5类别 圆形药片缺陷检测数据集VOC+YOLO格式330张3类别 新能源汽车充电插口类型识别检测数据集VOC+YOLO格式2486张3类别 运动鞋品牌检测数据集VOC+YOLO格式5824张6类别 西瓜叶子病害检测数据集VOC+YOLO格式1000张3类别 电力场景绝缘子串闪络污染破损检测数据集VOC+YOLO格式1600张3类别 智慧医疗肺部CT检测数据集VOC+YOLO格式4103张12类别 垃圾塑料袋检测数据集VOC+YOLO格式993张4类别 智慧医疗X光图像骨折严重程度检测数据集VOC+YOLO格式8000张5类别 地震后房屋建筑损坏程度分割数据集labelme格式1170张3类别 洋葱叶子病害检测数据集VOC+YOLO格式1899张7类别 印刷数字检测数据集VOC+YOLO格式1884张10类别 阀门检测数据集VOC+YOLO格式1647张1类别 辣椒叶子病害分类数据集2703张6类别 辣椒叶子病害检测数据集VOC+YOLO格式5000张4类别 辣椒叶子病害检测数据集VOC+YOLO格式874张3类别均为单个叶子 辣椒叶片叶子病害检测数据集VOC+YOLO格式1718张5类别 辣椒叶片叶子病害检测数据集VOC+YOLO格式2258张5类别 烟叶杂草检测数据集VOC+YOLO格式698张2类别 钻头缺陷检测数据集VOC+YOLO格式1969张9类别 棉花成熟度检测数据集VOC+YOLO格式4964张4类别(有漏标注) 电力场景配网绝缘子损坏检测数据集VOC+YOLO格式2384张1类别 盲道破损损坏检测数据集VOC+YOLO格式4195张1类别 茶叶叶片叶子品相识别检测数据集VOC+YOLO格式5631张2类别 田间大豆和杂草检测数据集VOC+YOLO格式1301张2类别 猫的情绪识别检测数据集VOC+YOLO格式4066张8类别 菊花蒲公英检测数据集VOC+YOLO格式1800张2类别 樱桃成熟度检测数据集VOC+YOLO格式6340张3类别 快递面单识别检测数据集VOC+YOLO格式5065张8类别 智能手机表面缺陷检测数据集VOC+YOLO格式1857张10类别 路桩状态识别检测数据集VOC+YOLO格式4227张2类别 智慧农业梨树叶子病害检测数据集VOC+YOLO格式3006张1类别 台阶垫斜坡垫检测数据集VOC+YOLO格式5783张1类别 水下管道树根检测数据集VOC+YOLO格式352张1类别 电路板芯片缺陷检测数据集VOC+YOLO格式4531张7类别 建筑物渗水漏水痕迹检测数据集VOC+YOLO格式1062张1类别 电力场景配网人员安全带识别检测数据集VOC+YOLO格式4651张2类别 建筑墙壁损伤缺陷检测数据集VOC+YOLO格式6872张19类别 草莓生长周期检测数据集VOC+YOLO格式2949张4类别 智慧医疗心脏超声图像间隔壁分割数据集labelme格式3092张1类别 口罩佩戴规范三分类检测数据集VOC+YOLO格式4946张3类别 苹果成熟度检测数据集VOC+YOLO格式2039张4类别 船舶类型检测数据集VOC+YOLO格式1177张5类别 番茄成熟度检测数据集VOC+YOLO格式1521张3类别 植物病虫害检测数据集VOC+YOLO格式7673张9类别 智慧医疗胃肠道息肉检测数据集VOC+YOLO格式3410张1类别 课堂行为数据集VOC+YOLO格式671张6类别 智慧医疗胃肠道息肉分割数据集labelme格式1000张1类别 智慧农业田间常见24种杂草检测数据集VOC+YOLO格式3095张24类别 柑橘叶子病害检测数据集VOC+YOLO格900张3类别 贝壳和鹅卵石分类数据集4250张2类别 智慧工地施工安全自动化监测检测数据集VOC+YOLO格式7164张10类别 电力场景电工验电断电操作规范检测数据集VOC+YOLO格式2084张6类别 智慧交通道路路面状态干燥潮湿分割数据集labelme格式1115张7类别 中国少数民族服饰分类数据集10320张56类别 中国少数民族服饰检测数据集VOC+YOLO格式2469张55类别 树上橘子检测数据集VOC+YOLO格式5215张1类别 噬菌体活性检测数据集VOC+YOLO格式1402张2类别 工业缺陷铸件工件缺陷检测数据集VOC+YOLO格式1445张7类别 膝关节损伤程度检测数据集VOC+YOLO格式7212张3类别 眼球疾病检测数据集VCO+YOLO格94张11类别 建筑物损坏程度分割数据集labelme格式2816张5类别 可回收垃圾检测数据集VOC+YOLO格式2516张6类别近距离拍摄 陶器陶瓷盘子缺陷检测数据集VOC+YOLO格式1399张3类别 智慧医疗宫腔镜图像病变检测数据集VOC+YOLO格式3381张8类别 布料布匹缺陷检测数据集VOC+YOLO格式1938张4类别 牛姿态检测数据集VOC+YOLO格式2750张6类别 渣土车检测数据集VOC+YOLO格式826张1类别 智慧工地施工工具检测数据集VOC+YOLO格式1869张49类别 多种蝴蝶识别分割数据集labelme格式784张9类别 液晶屏仪表文字ocr检测数据集VOC+YOLO格式4682张12类别 血压计OCR文字检测数据集VOC+YOLO格式2147张11类别 瓶盖缺陷检测数据集VOC+YOLO格式4516张11类别 酒瓶缺陷检测数据集VOC+YOLO格式2668张4类别 丝网印刷电路缺陷分类数据集3439张4类别 手扶电梯缺陷检测数据集VOC+YOLO格式67张1类别 快餐盘食物菜品检测数据集VOC+YOLO格式1027张73类别 超市商品检测数据集VOC+YOLO格式9498张105类别 医疗废弃物垃圾检测数据集VOC+YOLO格式2643张9类别 网球比赛检测数据集VOC+YOLO格式6648张5类别 夜晚场景下的人车检测数据集VOC+YOLO格式4199张6类别 测压表压力表计量表针头针尾检测数据集VOC+YOLO格式4862张4类别 蘑菇毒蘑菇检测数据集VOC+YOLO格式9247张14类别 城市道路车辆自行车摩托车公交车检测数据集VOC+YOLO格式5236张5类别 马铃薯叶子病害检测数据集VOC+YOLO格式1332张9类别 中式快餐食物检测数据集VOC+YOLO格式6675张36类别 田间常见农作物幼苗检测数据集VOC+YOLO格式7705张24类别 玉米苗和杂草检测数据集VOC+YOLO格式1250张2类别 玉米苗和杂草识别分割数据集labelme格式1997张3类别 显微镜下寄生虫卵类型检测数据集VOC+YOLO格式4400张11类别 水稻和杂草检测数据集VOC+YOLO格式1356张2类别 遥感图像建筑房屋树木水体分割数据集labelme格式2026张5类别 室内家具检测数据集VOC+YOLO格式2543张15类别 室内家具检测数据集VOC+YOLO格式7928张10类别 超声波图像阿尔兹海默症严重程度检测数据集VOC+YOLO格式3288张5类别 电力场景红外图像变电站液体泄漏检测数据集VOC+YOLO格式5034张1类别 田地杂草检测数据集VOC+YOLO格式4040张12类别 田间杂草检测数据集VOC+YOLO格式4790张14类别 显微镜下浮游藻类生物检测数据集VOC+YOLO格式16239张80类别 草莓成熟度检测数据集VOC+YOLO格式3294张4类别 毛桃病害分割数据集labelme格式212张6类别 毛桃病害检测数据集VOC+YOLO格式2780张5类别 货车上集装箱编号文字检测数据集VOC+YOLO格式1847张35类别 超声波图像甲状腺结节检测数据集VOC+YOLO格式1882张2类别 电力场景红外图像变电站目标检测数据集VOC+YOLO格式1175张13类别 天上飞行目标检测飞鸟飞机检测数据集VOC+YOLO格式3993张5类别 各种鱼类检测数据集VOC+YOLO格式2967张31类别 蟑螂检测数据集VOC+YOLO格式2051张1类别 排水管道裂缝腐蚀异常检测数据集VOC+YOLO格式3053张1类别 地下排水管道损害缺陷检测数据集VOC+YOLO格式2051张6类别 人行横道指示灯状态检测数据集VOC+YOLO格式4950张7类别 汽车表面划痕刮伤检测数据集VOC+YOLO格式1221张1类别 汽车表面划痕刮伤检测数据集VOC+YOLO格式1221张1类别 高质量工程车辆检测数据集VOC+YOLO格式5067张13类别 工程车辆检测数据集VOC+YOLO格式1239张10类别 自卸车和泵车检测数据集VOC+YOLO格式4863张2类别 车厢货物状态检测数据集VOC+YOLO格式2313张6类别 飞鸟小目标检测数据集VOC+YOLO格式1657张2类别 毛桃病害检测数据集VOC+YOLO格式142张4类别 煤矿场景下拖链检测数据集VOC+YOLO格式21407张1类别 煤矿场景下矿工行为检测数据集VOC+YOLO格式24709张8类别 煤矿场景下安全帽检测数据集VOC+YOLO格式20097张1类别 煤矿场景下的液压支撑角度异常检测数据集VOC+YOLO格式20024张9类别 煤矿场景下矿工检测数据集VOC+YOLO格式30691张1类别 煤矿场景下安全帽检测数据集VOC+YOLO格式179张2类别 煤矿传送带大煤块检测数据集VOC+YOLO格式20925张1类别 自然灾害检测数据集VOC+YOLO格式19151张4类别 遥感图像尾矿库检测数据集VOC+YOLO格式1183张1类别 煤矿井下钻场钻机卡盘煤矿工人安全帽检测数据集VOC+YOLO格式70677张5类别 木材缺陷检测数据集VOC+YOLO格式8961张10类别 木材缺陷分割数据集labelme格式1548张4类别 医疗显微图像结核杆菌检测数据集VOC+YOLO格式1218张1类别 残障人员物件检测数据集VOC+YOLO格式7385张6类别 医院照护和病人状态检测数据集VOC+YOLO格式2004张11类别 清洁员清扫状态检测数据集VOC+YOLO格式10330张3类别 航拍工程车辆检测数据集VOC+YOLO格式9121张4类别 X射线底片焊缝缺陷检测数据集VOC+YOLO格式3056张5类别 无人机视角道路缺陷损害损坏裂缝检测数据集VOC+YOLO格式2422张7类别 航拍工程车辆检测数据集VOC+YOLO格式1263张1类别 水稻穗检测数据集VOC+YOLO格式6038张1类别 海洋水下生物目标检测数据集VOC+YOLO格式9333张10类别 气体泄漏分割数据集labelme格式1612张1类别 遥感图像滑坡分类数据集2773张2类别 车站异常行为检测数据集VOC+YOLO格式2293张4类别 28种常见建筑风格分类图像数据集22975张28类别 电力场景隔离开关状态检测数据集VOC+YOLO格式600张2类别 电力场景输电线塔倒塌检测数据集VOC+YOLO格式4086张2类别 电力场景输电线高压铁塔检测数据集VOC+YOLO格式1023张1类别 电力场景红外测温图像绝缘套管分割数据集labelme格式2436张1类别 电力场景水泥电线杆缺陷检测数据集VOC+YOLO格式2052张11类别 链条缺陷检测数据集VOC+YOLO格式1422张7类别 电力场景螺栓螺帽销钉丢失检测数据集VOC+YOLO格式1494张5类别 电力场景绝缘子绑扎规范检测数据集VOC+YOLO格式1140张3类别 电力场景电力设备漏油检测数据集VOC+YOLO格式1114张36类别 电力场景变电站绝缘手套佩戴规范检测数据集VOC+YOLO格式2084张6类别 电力场景变电站火灾检测数据集VOC+YOLO格式3098张2类别 商店异常行为检测数据集VOC+YOLO格式9667张6类别 坦克检测数据集VOC+YOLO格式3469张1类别 智慧厨房不规范行为检测数据集VOC+YOLO格式7510张9类别 热成像老鼠检测数据集VOC+YOLO格式3309张1类别 电力场景输电杆塔关节检测数据集VOC+YOLO格式969张2类别 电力场景输电线电缆线灼伤烧伤破皮脱皮损伤缺陷检测数据集VOC+YOLO格式2054张1类别 稻田虫害识别分割数据集labelme格式7533张9类别 昆虫分割数据集labelme格式9484张1类别 老鼠分割数据集labelme格式1000张1类别 玻璃屏幕缺陷检测数据集VOC+YOLO格式2504张8类别 交通事故车辆分割数据集labelme格式3467张4类别 电力场景输电线覆冰分割数据集labelme格式1227张2类别 遥感图像塔吊检测数据集VOC+YOLO格式1567张1类 起重机检测数据集VOC+YOLO格式2815张1类 农田焚烧秸秆烟雾明火检测数据集VOC+YOLO格式7879张2类 遛狗不牵绳检测数据集VOC+YOLO格式12076张3类 电力巡检检测数据集VOC+YOLO格式9400张32类别 人字梯检测数据集VOC+YOLO格式2190张7类别 电路板导线缺陷检测数据集VOC+YOLO格式7367张9类别 人字梯检测数据集VOC+YOLO格式937张2类别 棉花杂质异物检测数据集VOC+YOLO格式1998张1类别 土豆病害检测数据集VOC+YOLO格式8908张5类别 特殊车辆检测数据集VOC+YOLO格式2730张3类别 辣椒病害检测数据集VOC+YOLO格式2161张2类别 电路板PCB缺陷检测数据集VOC+YOLO格式9666张8类别 齿轮缺陷检测数据集VOC+YOLO格式485张3类别 芯片引脚类型检测数据集VOC+YOLO格式582张5类别 芯片引脚缺陷检测数据集VOC+YOLO格式203张2类别 大豆叶子虫害检测数据集VOC+YOLO格式1794张12类别 泥石流检测数据集VOC+YOLO格式4732张3类别 红外图像火点检测数据集VOC+YOLO格式2510张1类别 螺栓松动丢失腐蚀生锈检测数据集VOC+YOLO格式504张4类别 衣物褶皱织物褶皱检测数据集VOC+YOLO格式939张1类别 电力场景输电路杆塔上的鸟巢检测数据集VOC+YOLO格式1000张1类别 电力场景输电线路异物检测数据集VOC+YOLO格式4370张1类别 电力场景配网缺陷系列之销钉缺失检测数据集VOC+YOLO格式3095张2类别 军事目标系列数据集地雷检测数据集VOC+YOLO格式1023张1类别 矿场工业传送带检测石块缺陷检测数据集VOC+YOLO格式2262张4类别 目标系列数据集导弹检测数据集VOC+YOLO格式969张1类别 目标系列数据集水下潜艇检测数据集VOC+YOLO格式428张1类别 目标系列数据集坦克装甲车检测数据集VOC+YOLO格式1464张4类别 钢材生锈铁生锈腐蚀识别分割数据集labelme格式3899张1类别 钢材缺陷识别分割数据集labelme格式693张4类别 钢材缺陷识别分割数据集labelme格式5597张4类别 天空分割数据集labelme格式1843张1类别 盲道识别及损坏分割数据集labelme格式995张2类别 头发分割数据集labelme格式3970张7类别 智慧交通道路积水分割数据集labelme格式8481张1类别 智慧交通道路积水检测数据集VOC+YOLO格式8474张1类别 乳腺癌分割数据集labelme格式165张3类别 水体分割数据集labelme格式2696张1类别 肺炎严重程度识别分割数据集labelme格式3071张3类别 肺部疾病分割数据集labelme格式3751张4类别 肺部疾病检测数据集VOC+YOLO格式1400张3类别 医学数据集肺肿瘤分割数据集labelme格式687张1类别 电力场景变压器检测数据集VOC+YOLO格式1971张13类别 电力场景电力部件热点区域检测数据集VOC+YOLO格式183张1类别 电力场景输电塔分割数据集labelme格式288张4类别 电力场景输电线覆冰检测数据集VOC+YOLO格式1983张3类别 传送带缺陷检测数据集VOC+YOLO格式1238张2类别 绳索绳子缺陷检测数据集VOC+YOLO格式1044张3类别 遥感图像光伏发电检测数据集VOC+YOLO格式271张1类别 遥感图像油罐检测数据集VOC+YOLO格式165张1类别 遥感图像电塔检测数据集VOC+YOLO格式244张1类别 遥感图像火电站烟囱检测数据集VOC+YOLO格式854张1类别 水表的数字表盘分割数据集labelme格式3023张13类别 水表的数字表盘分割数据集labelme格式3023张13类别 医学图像之图像分割数据集视神经青光眼分割数据集labelme格式903张2类别 医学分割数据集白内障严重程度分割数据集labelme格式719张3类别 医学分割数据集肾结石分割数据集labelme格式359张1类别 医学分割数据集肾肿瘤分割数据集labelme格式4099张2类别 医学分割数据集B超图像肾脏分割数据集labelme格式715张1类别 医学分割数据集B超图片肝脏分割数据集labelme格式271张1类别 光伏太阳能板灰尘识别分割数据集labelme格式2151张2类别 眼睛瞳孔和虹膜分割数据集labelme格式2662张2类别 舌头分割数据集labelme格式2557张1类别 图像分割数据集葡萄叶病害分割数据集labelme格式1375张3类别 图像分割数据集石头rock分割数据集labelme格式2602张3类别 橙子叶子病害分割数据集labelme格式1225张10类别 图像分割数据集植物图像叶片健康状态分割数据集labelme格式180张3类别 医学图像分割数据集脑肿瘤分割数据集labelme格式715张1类别 智慧城市道路分割数据集labelme格式2648张1类别 图像分割数据集牙齿分割牙齿病变分割数据集labelme格式2616张6类别 图像分割数据集海洋水体船只分割数据集labelme格式6123张3类别 医学图像分割数据集腹部肝脏多器官图像分割数据集labelme格式860张10类别 墙壁裂缝分割数据集labelme格式9192张1类别 电力场景输电线路与输电塔分割数据集1232张5类别 智慧工厂机械铸件缺陷检测数据集VOC+YOLO格式4270张8类别 笔记本电脑缺陷检测数据集VOC+YOLO格式1395张1类别 非酒精性脂肪肝NAFLD数据集csv文件3个.zip 人员持刀检测数据集VOC+YOLO格式9399张1类别 近距离刀棒检测数据集VOC+YOLO格式1751张3类别 西瓜病害分类数据集6930张4类别 小麦成熟度分类数据集10695张7类别 地质灾害自然灾害灾难分类数据集4868张12类 骨癌骨瘤检测检测数据集VOC+YOLO格式582张1类别 氧化锌避雷器破损缺陷检测数据集VOC+YOLO格式2408张1类别 光伏板鸟粪缺陷检测数据集VOC+YOLO格式1154张1类别 光伏板红外过热检测数据集VOC+YOLO格式1166张2类别 电力场景输电线路外力破环检测数据集VOC+YOLO格式5187张6类别 电力场景红外光伏板缺陷检测数据集VOC+YOLO格式2186张4类别 电力场景电气设备红外图像变压器检测数据集VOC+YOLO格式4271张14类别 遥感机场跑道检测数据集VOC+YOLO格式8116张2类别 桥梁损坏裂缝检测检测数据集VOC+YOLO格式2245张2类别 智慧农场航拍农田农机检测数据集VOC+YOLO格式4596张4类别 荔枝成熟度检测数据集VOC+YOLO格式1005张4类别 皮革缺陷检测数据集VOC+YOLO格式3077张3类别 智慧交通道路施工锥形桶路障检测数据集VOC+YOLO格式9454张5类别 车道线路面标识检测数据集VOC+YOLO格式4023张27类别 智慧交通城市道路红绿灯带转向检测数据集VOC+YOLO格式1079张8类别 轮毂缺陷检测数据集VOC+YOLO格式1445张7类别 健身动作检测数据集VOC+YOLO格式2827张6类别 内窥镜工业缺陷检测数据集VOC+YOLO格式2320张9类别 铁轨轨道配件检测数据集VOC+YOLO格式2905张7类别 牙齿CT健康状态检测数据集VOC+YOLO格式5040张6类别 应急车辆分类数据集应急车辆通常包括警车救护车和消防车1566张训练集+653张测试集 蚕茧缺陷检测数据集VOC+YOLO格式523张4类别 遥感滑坡检测数据集VOC+YOLO格式3588张1类别 电力场景变电站红外检测数据集VOC+YOLO格式6042张21类别 光伏无人机红外图像缺陷检测数据集VOC+YOLO格式419张10类别 海上救援检测数据集VOC+YOLO格式3429张6类别 茶叶叶龄检测数据集VOC+YOLO格式2195张4类别 大豆叶子虫害检测数据集VOC+YOLO格式1794张12类别 铁锈生锈检测数据集VOC+YOLO格式600张1类别 道路监控视角车辆检测数据集VOC+YOLO格式5236张5类别 非机动车检测数据集VOC+YOLO格式4141张4类别 非机动车未带安全帽检测数据集VOC+YOLO格式1000张4类别 水下鱼检测数据集VOC+YOLO格式2303张1类别 墙壁墙体发霉检测数据集VOC+YOLO格式2359张1类别 麻将检测数据集VOC+YOLO格式21814张34类别 道路交通拥挤检测数据集VOC+YOLO格式1899张1类别 拖鞋检测数据集VOC+YOLO格式779张1类别 车身缺陷检测数据集VOC+YOLO格式1071张4类别 乐器检测数据集VOC+YOLO格式843张12类别 药片检测数据集VOC+YOLO格式2729张3类别 珊瑚白化检测数据集VOC+YOLO格式2870张3类别 人头朝向检测数据集VOC+YOLO格式7970张10类别 车身朝向检测数据集VOC+YOLO格式906张8类别 盲道损坏检测数据集VOC+YOLO格式4195张1类别 钓鱼检测数据集VOC+YOLO格式1813张1类别 智慧交通火车站乘客上车物品遗落检测数据集VOC+YOLO格式2270张6类别 电梯扶梯跌倒行为检测数据集VOC+YOLO格式1529张3类别 探地雷达金属检测数据集VOC+YOLO格式1441张3类别 轴承表面缺陷检测数据集VOC+YOLO格式3945张3类别 睡岗检测数据集VOC+YOLO格式3292张4类别 公司员工行为检测数据集VOC+YOLO格式3853张3类别 智慧工地工地行为检测数据集VOC+YOLO格式7958张9类别 智慧工地工地扬尘检测数据集VOC+YOLO格式3382张1类别 智慧交通道路结冰检测数据集VOC+YOLO格式597张2类别 智慧交通城市道路打场晒粮检测数据集VOC+YOLO格式1065张1类别 公路落石滑坡坍塌倒树检测数据集VOC+YOLO格式1485张4类别 电力场景输电线腐蚀破损烧伤检测数据集VOC+YOLO格式363张1类别 高空远距离小目标航拍行人识别检测数据集VOC+YOLO格式7479张1类别 高空远距离小目标航拍人车自行车检测数据集VOC+YOLO格式8625张10类别 骨龄检测识别数据集VOC+YOLO+分类数据集+数据集介绍 脸部痤疮检测数据集VOC+YOLO格式3763张7类别 电力场景输电线覆冰结冰检测数据集VOC+YOLO格式148张1类别 电力场景输电线电缆缺陷检测数据集VOC+YOLO格式1167张8类别 电力场景越线闯入检测数据集VOC+YOLO格式258张1类别 电力场景各种部件检测数据集VOC+YOLO格式10559张18类别 污水排放检测数据集排污口检测数据集VOC+YOLO格式405张3类别 排污管道检测污水排放管道检测数据集VOC+YOLO格式10000张1类别 房屋冰凌冰锥冰柱检测数据集VOC+YOLO格式147张1类别 工程机械车辆挖掘机自卸卡车轮式装载机检测数据集VOC+YOLO格式2644张3类别 天气识别分类数据集6862张11类别 基于yolov10的烟雾明火检测森林火灾系统python源码+pytorch模型+评估指标曲线+精美GUI界面+数据集 [数据集][目标检测]鸡粪疾病检测数据集VOC+YOLO格式15589张4类别 [数据集][目标检测]鱼病害检测数据集VOC+YOLO格式8474张4类别 [数据集][目标检测]遥感卫星图雷达天线检测数据集VOC+YOLO格式215张1类别 [数据集][目标检测]道路缺陷数据集VOC+YOLO格式5000张8类别 [数据集][目标检测]过敏原食物检测数据集VOC+YOLO格式14659张30类别 [数据集][目标检测]行人乱丢垃圾检测数据集VOC+YOLO格式3264张2类别 [数据集][目标检测]葡萄叶病害检测数据集VOC+YOLO格式5015张4类别 [数据集][目标检测]苹果缺陷检测数据集VOC+YOLO格式6970张4类别 [数据集][目标检测]苦瓜检测数据集VOC+YOLO格式1169张1类别 [数据集][目标检测]花粉数据集花粉粒检测数据集VOC+YOLO格式2412张20类别 [数据集][目标检测]矿区卡车检测数据集VOC+YOLO格式989张2类别 [数据集][目标检测]电力场景变电站吸烟检测数据集VOC+YOLO格式578张1类别 [数据集][目标检测]猫行为检测数据集VOC+YOLO格式5997张5类别 [数据集][目标检测]牙齿健康状态检测数据集VOC+YOLO格式2792张3类别 [数据集][目标检测]洪水灾害场景车辆行人淹水检测数据集VOC+YOLO格式702张12类别 [数据集][目标检测]水上浮标检测数据集VOC+YOLO格式1163张2类别 [数据集][目标检测]智慧工地矿区安全帽检测数据集VOC+YOLO格式20097张1类别 [数据集][目标检测]智慧工厂流水钱水泥袋检测数据集VOC+YOLO格式1787张1类别 [数据集][目标检测]智慧城市-交通违规行为检测数据集VOC+YOLO格式4662张7类别 [数据集][目标检测]智慧厨房菜品识别检测数据集VOC+YOLO格式5790张16类别 [数据集][目标检测]手写数字检测数据集VOC+YOLO格式5013张10类别 [数据集][目标检测]座椅缺陷检测数据集VOC+YOLO格式1268张4类别 [数据集][目标检测]墙面墙体损伤等级检测数据集VOC+YOLO格式4629张4类别 [数据集][目标检测]基于yolov5增强数据集算法mosaic来扩充数据集自动生成增强图片和对应标注python源码 [数据集][目标检测]厨房食品佐料检测数据集VOC+YOLO格式602张18类别 [数据集][目标检测]人体行为检测数据集VOC+YOLO格式9097张17类别 [数据集][目标检测]云层类型检测数据集VOC+YOLO格式3319张5类别 [数据集][目标检测]X光图口腔结构检测数据集VOC+YOLO格式2297张6类别 [数据集][图像分类]黄瓜及其叶子病害分类数据集6400张8类别 [数据集][图像分类]智慧厨房菜品识别分类数据集10285张8类别 [数据集][图像分类]智慧农业农作物识别分类数据集829张30类别 ATM机旁危险物品检测数据集VOC+YOLO格式1251张5类别 [数据集汇总]智慧交通-铁路相关数据集汇总 电力行业电气领域相关数据集下载地址汇总输电线路变电站电网应用数据集汇总(全网最全) 2024年图像分类数据集大合集所有下载地址汇总 2024年目标检测数据集大合集所有下载地址汇总 [数据集][目标检测]电力场景输电线路巡检检测数据集VOC+YOLO格式8667张50类别 [数据集][目标检测]牛行为检测数据集VOC+YOLO格式2922张4类别 智慧城市街道涂鸦垃圾故障路灯市容检测数据集VOC+YOLO格式19263张11类别 工程机械车辆挖掘机自卸卡车轮式装载机检测数据集VOC+YOLO格式2644张3类别 [数据集][目标检测]电力场景防震锤缺陷检测数据集VOC+YOLO格式705张1类别 [数据集][目标检测]猪数据集VOC-2856张 [数据集][目标检测]辣椒缺陷检测数据集VOC+YOLO格式695张5类别 [数据集][目标检测]海上红外目标检测检测数据集VOC+YOLO格式8402张7类别 [数据集][图像分类]水果分类数据集22万张262类别 [数据集][目标检测]Udacity交通目标检测数据集VOC+YOLO格式13063张11类别 [数据集][目标检测]中国交通标志TT100K检测数据集VOC+YOLO格式7962张45类别 [数据集][目标检测]DOTAv2.0遥感航拍目标检测数据集VOC+YOLO格式4840张16类别 [数据集][目标检测]DOTAv1.0遥感航拍目标检测数据集VOC+YOLO格式1713张16类别 [数据集][目标检测]外部墙体损伤缺陷检测外墙损坏等级检测数据集VOC+YOLO格式4629张4类别 [数据集][目标检测]水泥外墙缺陷检测数据集VOC+YOLO格式2947张4类别 [数据集][目标检测]PCB电路板元件检测数据集VOC+YOLO格式3948张29类别 [数据集][目标检测]手机识别检测数据集VOC+YOLO格式9997张1类别 [数据集][目标检测]中草药类型识别检测数据集VOC+YOLO格式7976张45类别 [数据集][目标检测]蘑菇类型识别检测数据集VOC+YOLO格式8430张21类别 [数据集][目标检测]俯视场景航拍小目标三轮车遮阳伞三轮车检测数据集VOC+YOLO格式5756张3类别 [数据集][目标检测]航拍路面直行和转弯标志检测数据集VOC+YOLO格式566张9类别 [数据集][目标检测]不同颜色的安全帽检测数据集VOC+YOLO格式7574张5类别 [数据集][目标检测]DMS驾驶员抽烟打电话喝水吃东西检测数据集VOC+YOLO格式5743张9类别 [数据集][目标检测]文本表格检测数据集VOC+YOLO格式6688张5类别 [数据集][目标检测]智慧养殖场肉鸡目标检测数据集VOC+YOLO格式3548张1类别 [数据集][目标检测]智慧养殖场肉鸡健康状态检测数据集VOC+YOLO格式4657张2类别 [数据集][目标检测]红外微小目标无人机直升机飞机飞鸟检测数据集VOC+YOLO格式7559张4类别 [数据集][目标检测]无人机飞鸟检测数据集VOC+YOLO格式6647张2类别 [数据集][目标检测]棉花叶子病害检测数据集VOC+YOLO格式977张22类别 [数据集][目标检测]战斗机检测数据集VOC+YOLO格式10100张43类别 [数据集][目标检测]茶叶病害检测数据集VOC+YOLO格式9591张8类别 [数据集][图像分类]茶叶病害分类数据集6749张7类别 [数据集][目标检测]混凝土缺陷检测数据集VOC+YOLO格式7513张7类别 [数据集][目标检测]无人机识别检测数据集VOC+YOLO格式6986张1类别 [数据集][图像分类]痤疮严重程度分级分类数据集999张3类别 [数据集][目标检测]智慧交通铁路人员危险行为躺站坐检测数据集VOC+YOLO格式3766张4类别 [数据集][目标检测]智慧交通铁轨裂缝检测数据集VOC+YOLO格式4类别 [数据集][目标检测]智慧交通铁路异物入侵检测数据集VOC+YOLO格式802张7类别 [数据集][目标检测]葡萄成熟度检测数据集VOC+YOLO格式1123张3类别 [数据集][目标检测]疟疾恶性疟原虫物种目标检测数据集VOC+YOLO格式948张1类别 [数据集][目标检测]俯拍航拍森林火灾检测数据集VOC+YOLO格式6116张2类别 [数据集][目标检测]车油口挡板开关闭合检测数据集VOC+YOLO格式138张2类别 [数据集][目标检测]车窗状态检测车窗开关检测数据集VOC+YOLO格式299张3类别 [数据集][目标检测]男女性别检测数据集VOC+YOLO格式9769张2类别 [数据集][图像分类]不同年龄段男女年龄区间分类数据集7981张12类别 [数据集][目标检测]岩石种类检测数据集VOC+YOLO格式4766张9类别 [数据集][目标检测]脊椎检测数据集VOC+YOLO格式1137张1类别 数据集VOC格式打电话检测数据集-5364张 [数据集][目标检测]学生课堂行为检测数据集VOC+YOLO格式5622张7类别 [数据集][目标检测]烟叶病害检测数据集VOC+YOLO格式612张3类别 [数据集][目标检测]高铁受电弓检测数据集VOC+YOLO格式1245张2类别 [数据集][目标检测]汽车头部尾部检测数据集VOC+YOLO格式5319张3类别 [数据集][目标检测]遗失物遗落物检测数据集VOC+YOLO格式2173张52类别 [数据集][目标检测]乱堆物料检测数据集VOC+YOLO格式1143张1类别 [数据集][目标检测]河道垃圾检测数据集VOC+YOLO格式2274张8类别 挖掘机叉车工程车辆检测数据集VOC+YOLO格式5067张7类别 [数据集][目标检测]打电话检测数据集VOC+YOLO格式8985张1类别 [数据集][图像分类]眼睛睁开闭合分类数据集2361张2类别 [数据集][图像分类]嘴巴张开闭合分类数据集6397长2类别 [数据集][目标检测]百事可乐可口可乐瓶子检测数据集VOC+YOLO格式195张2类别 [数据集][图像分类]35种蛇分类数据集13185张35类别 [数据集][图像分类]熊分类数据集309张5类别黑熊泰迪北极熊等 [数据集][目标检测]肺炎检测数据集VOC+YOLO格式4983张2类别 [数据集][目标检测]血细胞检测数据集VOC+YOLO格式2757张4类别 [数据集][目标检测]苹果叶病害检测数据集VOC+YOLO格式5867张13类别 [数据集][目标检测]智慧农业草莓叶子病虫害检测数据集VOC+YOLO格式4040张9类别 [数据集][目标检测]抽烟检测数据集VOC+YOLO格式22559张2类别 [数据集][目标检测]卫星遥感舰船检测数据集VOC+YOLO格式2238张17类别 [数据集][目标检测]人脸口罩佩戴目标检测数据集VOC+YOLO格式8068张3类别 [数据集][目标检测]井盖丢失未盖破损检测数据集VOC+YOLO格式2890张5类别 [数据集][目标检测]电动车头盔佩戴检测数据集VOC+YOLO格式4235张5类别 [数据集][目标检测]电动车入梯进电梯电单车入梯检测数据集VOC+YOLO格式7106张3类别 [数据集][目标检测]电梯内广告牌电动车检测数据集VOC+YOLO格式2787张4类别 [数据集][目标检测]西红柿缺陷检测数据集VOC+YOLO格式17318张3类别 [数据集][目标检测]西红柿成熟度检测数据集VOC+YOLO格式3241张5类别 [数据集][目标检测]水面垃圾检测数据集VOC+YOLO格式2027张1类别 [数据集][目标检测]车身部件损坏车体缺陷检测数据集VOC+YOLO格式7825张15类别 [数据集][目标检测]翻越栏杆行为检测数据集VOC+YOLO格式512张1类别 [数据集][目标检测]机油泄漏检测数据集VOC+YOLO格式43张1类别 [数据集][目标检测]汽油泄漏检测数据集VOC+YOLO格式237张2类别 [数据集][目标检测]石油泄漏检测数据集VOC+YOLO格式6633张1类别 [数据集][目标检测]轮胎缺陷检测数据集VOC+YOLO格式2154张4类别 [数据集][目标检测]轮胎检测数据集VOC+YOLO格式4629张1类别 [数据集][目标检测]鲜花检测数据集VOC+YOLO格式25215张106类别 [数据集][目标检测]街道乱放广告牌检测数据集VOC+YOLO格式114张1类别 [数据集][目标检测]街道乱堆垃圾检测数据集VOC+YOLO格式94张1类别 [数据集][目标检测]街头摊贩识别检测数据集VOC+YOLO格式758张1类别 [数据集][目标检测]智慧牧场猪只检测数据集VOC+YOLO格式16245张1类别 [数据集][目标检测]灭火器检测数据集VOC+YOLO格式3255张1类别 [数据集][目标检测]安检x光危险物品识别检测数据集VOC+YOLO格式9551张12类别 [数据集][目标检测]玉米病害检测数据集VOC+YOLO格式6000张4类别 [数据集][目标检测]课堂行行为检测数据集VOC+YOLO格式4065张12类别 全国初中高中小学学校名单2024年9月版 [数据集][目标检测]玻璃瓶塑料瓶检测数据集VOC+YOLO格式8943张2类别 [数据集][目标检测]直肠息肉病变检测数据集VOC+YOLO格式10725张6类别 [数据集][目标检测]电力场景输电线均压环歪斜检测数据集VOC+YOLO格式303张2类别 [数据集][目标检测]管道漏水泄漏破损检测数据集VOC+YOLO格式2614张4类 [数据集][目标检测]电力场景输电线悬垂线夹检测数据集VOC+YOLO格式2538张2类别 [数据集][目标检测]电力场景输电线防震锤检测数据集VOC+YOLO格式2721张2类别 [数据集][目标检测]电力场景输电线导线散股检测数据集VOC+YOLO格式3890张1类别 [数据集][目标检测]风力发电机叶片损伤检测数据集VOC+YOLO格式5029张8类别 [数据集][目标检测]电力场景红外图像输电线路绝缘子检测数据集VOC+YOLO格式1846张1类别 [数据集][目标检测]考场行为作弊检测数据集VOC+YOLO格式4413张4类别 [数据集][目标检测]光伏发电板红外图像鸟粪检测数据集VOC+YOLO格式173张1类别 [数据集][目标检测]电力场景输电线杆塔塔架金属锈蚀腐蚀生锈检测数据集VOC+YOLO格式1344张1类别 [数据集][目标检测]电力场景输电线异物检测数据集VOC+YOLO格式2060张1类别 [数据集][目标检测]流水线物件检测数据集VOC+YOLO格式9255张26类别 [数据集][目标检测]建筑工地楼层空洞检测数据集VOC+YOLO格式2588张1类别 [数据集][目标检测]集装箱缺陷检测数据集VOC+YOLO格式4127张3类别 [数据集][目标检测]快递包裹检测数据集VOC+YOLO格式5382张1类别 [数据集][目标检测]街灯路灯检测数据集VOC+YOLO格式1893张1类别 [数据集][目标检测]道路积水检测数据集VOC+YOLO格式2699张1类别 [数据集][目标检测]航拍屋顶检测数据集VOC+YOLO格式458张3类别 [数据集][目标检测]停车场空位检测数据集VOC+YOLO格式7959张2类别 [数据集][目标检测]agvs仓储机器人检测数据集VOC+YOLO格式967张3类别 [数据集][目标检测]瞳孔虹膜检测数据集VOC+YOLO格式8768张2类别 [数据集][目标检测]工程机械车辆检测数据集VOC+YOLO格式3189张10类别 [数据集][目标检测]木材缺陷检测数据集VOC+YOLO格式2383张10类别 [数据集][目标检测]铁轨缺陷检测数据集VOC+YOLO格式4020张4类别 [数据集][目标检测]竹子甘蔗发芽缺陷检测数据集VOC+YOLO格式2953张3类别 [数据集][目标检测]夜间老鼠检测数据集VOC+YOLO格式316张1类别+视频文件1个 [数据集][目标检测]扳手检测数据集VOC+YOLO格式1042张1类别 [数据集][目标检测]绳子检测数据集VOC+YOLO格式322张1类别 [数据集][目标检测]锤子检测数据集VOC+YOLO格式1510张1类别 [数据集][目标检测]起子检测数据集VOC+YOLO格式1215张1类别 [数据集][目标检测]手钳检测数据集VOC+YOLO格式141张1类别 [数据集][目标检测]机械常用工具检测数据集VOC+YOLO格式4713张8类别 [数据集][目标检测]电力场景轭式悬架锈蚀分类数据集6351张2类别 [数据集][图像分类]电力场景电力线固定处连接处腐蚀有鸟巢分类数据集1279张3类别 [数据集][图像分类]电力场景聚合物绝缘子上卸扣腐蚀分类数据集1546张2类别 [数据集][图像分类]电力场景避雷针悬挂处腐蚀分类数据集909张2类别 [数据集][图像分类]波色绝缘子缺失分类数据集1440张2类别 [数据集][图像分类]超声波肾脏结石分类数据集9416张2类别 [数据集][图像分类]肾脏病变分类数据集识别囊肿肿瘤结石数据集11756张4类别 [数据集][目标检测]违规撑伞检测数据集VOC+YOLO格式341张2类别 [数据集][目标检测]太阳能光伏板积灰灰尘检测数据集VOC+YOLO格式1463张1类别 [数据集][目标检测]轴承缺陷划痕检测数据集VOC+YOLO格式1166张1类别 [数据集][目标检测]肾结石检测数据集VOC+YOLO格式1299张1类别 [数据集][目标检测]辣椒病害成熟度检测数据集VOC+YOLO格式2748张5类别 [数据集][目标检测]船上翻越栏杆危险行为检测数据集VOC+YOLO格式3678张1类别 [数据集][目标检测]生产线上金属罐易拉罐正反面检测数据集VOC+YOLO格式2715张2类别 [数据集][目标检测]易拉罐底部缺陷检测数据集VOC+YOLO格式1122张5类别 [数据集][目标检测]金属罐缺陷检测数据集VOC+YOLO格式8095张4类别 [数据集][目标检测]蝗虫检测数据集VOC+YOLO格式1501张1类别 [数据集][目标检测]躺坐站识别检测数据集VOC+YOLO格式9488张3类别 [数据集][目标检测]野猪检测数据集VOC+YOLO格式1000张1类别 [数据集][目标检测]婴儿车检测数据集VOC+YOLO格式1073张5类别 [数据集][目标检测]拐杖检测数据集VOC+YOLO格式638张1类别 [数据集][目标检测]拐杖检测数据集VOC+YOLO格式2778张1类别 [数据集][目标检测]导盲犬拐杖检测数据集VOC+YOLO格式4635张2类别 [数据集][VOC][202206][重制版]烟火数据集烟雾明火2类别数据集VOC-6460张 黑色矩形块检测数据集VOC+YOLO格式2000张1类别 电表读数检测数据集VOC+YOLO格式18156张12类别 绝缘子陶瓷绝缘子玻色绝缘子聚合物绝缘子检测数据集VOC+YOLO格式2050张3类别 [数据集][目标检测]护目镜检测数据集VOC+YOLO格式888张1类别 [数据集][目标检测]刀具匕首持刀检测数据集VOC+YOLO格式8810张1类别 [数据集][目标检测]轮椅检测数据集VOC+YOLO格式13826张1类别 [数据集][目标检测]螺丝缺陷检测数据集VOC+YOLO格式3081张2类别 [数据集][目标检测]螺丝螺母检测数据集VOC+YOLO格式2400张2类别 [数据集][目标检测]建筑工地场景安全帽手套防护服检测数据集VOC+YOLO格式8845张14类别 [数据集][目标检测]桥梁检测数据集VOC+YOLO格式1116张1类别 [数据集][目标检测]围栏破损检测数据集VOC+YOLO格式1196张1类别 [数据集][目标检测]睡岗检测数据集VOC+YOLO格式3316张4类别 [数据集][目标检测]人员状态跑睡抽烟打电话跌倒检测数据集4943张5类别 [数据集][目标检测]猪只状态吃喝睡站检测数据集VOC+YOLO格式530张4类别 [数据集][目标检测]婴儿状态睡觉哭泣检测数据集VOC+YOLO格式7109张3类别 [数据集][目标检测]水面垃圾水面漂浮物检测数据集VOC+YOLO格式3749张1类别 [数据集][目标检测]井盖未盖好检测数据集VOC+YOLO格式20123张2类别 [数据集][目标检测]城市街道井盖破损未盖丢失检测数据集VOC+YOLO格式4404张5类别 [数据集][目标检测]金属架螺栓螺丝有无检测数据集VOC+YOLO格式857张3类别 [数据集][目标检测]游泳者溺水检测数据集VOC+YOLO格式4599张2类别 [数据集][目标检测]游泳者溺水检测数据集VOC+YOLO格式8275张4类别 [数据集][目标检测]电缆钢丝绳线缆缺陷检测数据集VOC+YOLO格式1800张3类别 [数据集][目标检测]电力场景下电柜箱门把手检测数据集VOC+YOLO格式1167张1类别 [数据集][目标检测]鸡蛋缺陷检测数据集VOC+YOLO格式2918张2类别 电梯开关状态人员进出检测数据集VOC+YOLO格式2220张4类别 [数据集][目标检测]斑马线人行横道检测数据集VOC+YOLO格式793张1类别 [数据集][目标检测]棉花检测数据集VOC+YOLO格式389张1类别 [数据集][目标检测]花生米计数霉变检测数据集VOC+YOLO格式387张2类别 中国车牌检测数据集VOC+YOLO格式2001张1类别 [数据集][目标检测]驾驶中眼睛疲劳检测数据集VOC+YOLO格式4030张2类别 [数据集][目标检测]药片药丸检测数据集VOC+YOLO格式152张1类别 [数据集][图像分类]瑜伽动作分类数据集1238张5类别 [数据集][目标检测]棉花叶子害虫检测数据集VOC+YOLO格式595张1类别 棉花叶子病害分类数据集3601张6类别 鞋子分类数据集17399张69类别 [数据集][目标检测]减速带检测数据集VOC+YOLO格式5400张1类别 [数据集][实例分割]减速带分割数据集json+yolo格式5400张1类别 [数据集][目标检测]减速区域检测数据集VOC+YOLO格式1654张1类别 [数据集][目标检测]cs反恐精英检测数据集VOC+YOLO格式204张4类别 [数据集][目标检测]胸部解剖检测数据集VOC+YOLO格式100张10类别 [数据集][目标检测]中国象棋检测数据集VOC+YOLO格式300张12类别 [数据集][目标检测]变电站火灾检测电力场景烟雾明火检测数据集VOC+YOLO格式140张2类别真实场景非PS合成 [数据集][图像分类]人种黄种人白人黑人等分类数据集56000张7类别 [数据集][图像分类]人种黄种人白人黑人分类数据集970张4类别 玉米粒计数检测数据集VOC+YOLO格式107张1类别 人体部位眼耳手腿分类数据集4376张4类别 [数据集][图像分类]黑色素瘤分类数据集10015张7类别 [数据集][目标检测]足球运动员检测数据集VOC+YOLO格式11124张2类别 [数据集][目标检测]足球场足球运动员身份识别足球裁判员数据集VOC+YOLO格式312张4类别 [数据集][目标检测]橙子橘子桔子病害检测数据集VOC+YOLO格式2814张4类别 橘子叶子病害分类数据集38432张5类别 开源目标检测数据集汇总 widerface人脸检测数据集VOC+YOLO格式16094张1类别 [数据集][目标检测]厨房积水检测数据集VOC+YOLO格式88张2类别 [数据集][目标检测]路边倒树检测数据集VOC+YOLO格式1238张2类别 [数据集][图像分类]城市异常情况路边倒树火灾水灾交通事故分类数据集15223张8类别 [数据集][目标检测]攀墙攀越墙壁数据集VOC格式-701张 [数据集][图像分类]十二生肖分类数据集8492张12类别 [数据集][目标检测]电力场景电表类型检测数据集VOC+YOLO格式3232张5类别 [数据集][目标检测]叶子计数检测数据集VOC+YOLO格式240张1类别 [数据集][目标检测]盲道检测数据集VOC+YOLO格式2173张1类别 [数据集][目标检测]道路圆石墩检测数据集VOC+YOLO格式461张1类别 [数据集][目标检测]医疗防护服检测数据集VOC+YOLO格式649张7类别 [数据集][图像分类]煤矿分类数据集351张4类别 [数据集][图像分类]茶叶叶子病害分类数据集304张4类别 [数据集][图像分类]蘑菇分类数据集3122张215类别 [数据集][图像分类]蘑菇分类数据集14689张50类别 [数据集][目标检测]室内积水检测数据集VOC+YOLO格式761张1类别 [数据集][目标检测]csgo头部身体检测数据集VOC+YOLO格式1265张4类别 [数据集][目标检测]远距离小目标仰拍无人机检测数据集VOC+YOLO格式10672张1类别 [数据集][目标检测]电力工地场景下的人头检测数据集VOC+YOLO格式7035张1类别 [数据集][图像分类]白内障分类数据集601张4类别 [数据集][目标检测]塑料纸张垃圾袋检测数据集VOC+YOLO格式1.5w张3类别 [数据集][目标检测]40种飞机检测数据集VOC+YOLO格式15199张40类别 [数据集][目标检测]芒果害虫检测数据集VOC+YOLO格式3575张10类别 [数据集][目标检测]五种猴子种类检测数据集VOC+YOLO格式649张5类别 [数据集][图像分类]骨关节炎严重程度分类数据集14038张4分类 [数据集][目标检测]手枪检测数据集VOC+YOLO格式3000张1类别 [数据集][目标检测]剪刀石头布检测数据集VOC+YOLO格式1973张3类别 [数据集][目标检测]水下管道泄漏破损检测数据集VOC+YOLO格式2069张2类别 [数据集][目标检测]焊接处缺陷检测数据集VOC+YOLO格式3400张8类别 [数据集][目标检测]脑溢血检测数据集VOC+YOLO格式767张2类别 [数据集][目标检测]吉他检测数据集VOC+YOLO格式66张1类别 [数据集][目标检测]猕猴桃检测数据集VOC+YOLO格式1838张1类别 [数据集][目标检测]老鼠检测数据集VOC+YOLO格式4107张1类别 [数据集][目标检测]旋风检测数据集VOC+YOLO格式157张1类别 [数据集][目标检测]石榴成熟度检测数据集VOC+YOLO格式5857张5类别 [数据集][目标检测]糖尿病肾病检测数据集VOC+YOLO格式4122张5类别 [数据集][目标检测]课堂行为举手阅读睡觉转头朝前看检测数据集VOC+YOLO格式2262张5类别 [数据集][目标检测]脑肿瘤检测数据集VOC+YOLO格式9787张3类别 [数据集][图像分类]轮胎纹理识别裂纹和正常轮胎分类数据集1028张2类别 [数据集][目标检测]轮胎检测数据集VOC+YOLO格式439张1类别 [数据集][目标检测][数据集][目标检测]智能手机检测数据集VOC格式5447张 [数据集][目标检测]数据集VOC格式岸边垂钓钓鱼fishing目标检测数据集-4330张 [数据集][目标检测]猫狗检测数据集VOC+YOLO格式8291张2类别 [数据集][图像分类]家庭场景下的家具分类数据集1010张101类别 骨折检测数据集VOC+YOLO格式717张1类别 骨折分类数据集1129张10类别 [数据集][目标检测]航空发动机缺陷检测数据集VOC+YOLO格式291张4类别 [数据集][目标检测]RSNA肺炎检测数据集VOC+YOLO格式6012张1类别 [数据集][目标检测]喝水检测数据集VOC+YOLO格式995张3类别 [数据集][目标检测]红外车辆检测数据集VOC+YOLO格式13979张类别 [数据集][目标检测]红外兔子检测数据集VOC+YOLO格式96张1类别 [数据集][目标检测]红外海洋船只检测数据集VOC+YOLO格式8402张7类别 红外成像人员检测数据集VOC+YOLO格式5838张1类别 [数据集][目标检测]红外人狗检测数据集VOC+YOLO格式185张2类别 [数据集][目标检测]打架检测数据集VOC格式3146张2类别 [数据集][目标检测]道路井盖下水道井盖开关闭和检测数据集VOC+YOLO格式407张2类别 [数据集][目标检测]伤口检测数据集VOC+YOLO格式2760张1类别 [数据集][目标检测]抽烟吸烟检测数据集VOC+YOLO格式1449张1类别 高空无人机拍摄的车辆检测数据集VOC+YOLO格式1577张1类别 [数据集][目标检测]车辆类型检测数据集VOC+YOLO格式8144张196类别 [数据集][图像分类]车辆分类数据集1600张10类别 烟雾明火检测数据集VOC+YOLO格式10000张2类别 [数据集][目标检测]森林火灾检测数据集VOC+YOLO格式362张1类别 刀棒识别检测数据集VOC+YOLO格式401张2类别 [数据集][目标检测]手枪机枪刀检测数据集VOC+YOLO格式5990张3类别 [数据集][图像分类]11类食物分类数据集13296张11类别 [数据集][目标检测]超市秤盘水果检测数据集VOC+YOLO格式4592张14类别 [数据集][目标检测]鱼头鱼尾检测数据集VOC+YOLO格式200张2类别 [数据集][目标检测]抽烟喝酒检测数据集VOC+YOLO格式1026张2类别 [数据集][目标检测]番茄西红柿叶子病害检测数据集VOC+YOLO格式725张7类别 [数据集][目标检测]痤疮检测数据集VOC+YOLO格式915张1类别 [数据集][目标检测]风力涡轮机缺陷检测数据集VOC+YOLO格式2992张2类别 [数据集][图像分类]狗脸分类数据集18247张1174类别 [数据集][目标检测]狗脸检测数据集VOC+YOLO格式6154张1类别 [数据集][目标检测]危险驾驶行为驾驶员状态分心喝水疲劳驾驶检测数据集VOC+YOLO格式14855张6类别 [数据集][目标检测]金属瓶盖瑕疵检测数据集VOC+YOLO格式1609张1类别 [数据集][目标检测]瓶装酒酒盖液体瑕疵缺陷检测数据集VOC+YOLO格式5031张13类别 [数据集][目标检测]圆三角形矩形检测数据集VOC+YOLO格式228张3类别 [数据集][目标检测]电力场景电力目标检测数据集VOC+YOLO格式476张5类别 [数据集][目标检测]卡车抓斗卸车检测数据集VOC+YOLO格式213张2类别 [数据集][目标检测]弹簧上料检测数据集VOC+YOLO格式142张2类别 [数据集][目标检测]结直肠息肉内镜图像病变检测数据集13524张2类别 [数据集][目标检测]肺结节检测数据集VOC+YOLO格式1186张1类别 [数据集][目标检测]蕃茄核桃桔子龙眼青枣5种水果检测数据集VOC+YOLO格式270张5类别 [数据集][图像分类]杂草分类数据集17509张9类别 [数据集][目标检测]道路交通红绿灯检测数据集VOC+YOLO格式5782张5类别 [数据集][图像分类]抽烟打电话分类数据集6150张3类别 [数据集][目标检测]手扶电梯地铁电梯缺陷检测数据集VOC+YOLO格式67张2类别 [数据集][目标检测]电力场景鸟巢垃圾风筝气球检测数据集VOC+YOLO格式1300张4类别 [数据集][目标检测]电力场景遥感输电杆塔检测数据集VOC+YOLO格式400张1类别 [数据集][目标检测]电力场景安全帽检测数据集VOC+YOLO格式295张2类别 电力场景设备漏油检测数据集VOC+YOLO格式338张1类别 [数据集][目标检测]垃圾检测数据集VOC+YOLO格式1814张5类别 船舶检测数据集VOC+YOLO格式7000张6类别 [数据集][目标检测]纸箱子检测数据集VOC+YOLO格式8375张1类别 [数据集][目标检测]管道焊缝质量检测数据集VOC+YOLO格式1134张2类别 [数据集][目标检测]光栅检测数据集VOC+YOLO格式153张1类别 [数据集][目标检测]交通标志检测数据集VOC+YOLO格式877张4类别 [数据集][目标检测]交通灯检测数据集VOC+YOLO格式2600张1类别 [数据集][目标检测]手检测数据集VOC+YOLO格式1769张1类别 [数据集][目标检测]手势识别检测数据集VOC+YOLO格式778张7类别 [数据集][目标检测]狗鼻子检测数据集VOC+YOLO格式3099张1类别 小麦穗检测数据集VOC+YOLO格式6508张1类别 流水线包裹检测数据集VOC+YOLO格式145张4类别 苹果香蕉橙子菠萝葡萄西瓜水果检测识别数据集VOC+YOLO格式8475张6类别 课堂学生学习状态检测数据集VOC+YOLO格式8884张8类别 香蕉新鲜度等级识别香蕉成熟度识别分类数据集13478张4类别 农作物害虫检测数据集VOC+YOLO格式3575张10类别 农作物害虫检测数据集VOC+YOLO格式18975张97类别 农作物害虫分类数据集12846张27类别 桑叶虫害检测数据集VOC+YOLO格式1787张6类别 对虾病害分类数据集889张7类别 稻谷叶子虫害检测数据集VOC+YOLO格式1566张9类别 [数据集][图像分类]道路交通事故分类数据集1070张4类别 [数据集][目标检测]道路交通事故检测数据集VOC+YOLO格式11819张2类别 [数据集][图像分类]自然灾害分类数据集13306张8类别 [数据集][目标检测]公共场所危险物品检测数据集VOC+YOLO格式1431张6类别 [数据集][目标检测]道路行人车辆坑洞锥形桶检测数据集VOC+YOLO格式6275张4类别 [数据集][图像分类]河道污染分类数据集1923张4类别 [数据集][目标检测]麻雀检测数据集VOC+YOLO格式1157张1类别 [数据集][目标检测]高质量铁路轨道缺陷检测数据集VOC+YOLO格式1050张6类别 [数据集][目标检测]番茄成熟度检测数据集VOC+YOLO格式277张3类别 [数据集][目标检测]铁路工人工服安全帽检测数据集VOC+YOLO格式3065张3类别 [数据集][目标检测]焊接件表面缺陷检测数据集VOC+YOLO格式2292张10类别 [数据集][目标检测]铝片表面工业缺陷检测数据集VOC+YOLO格式400张4类别 [数据集][目标检测]草莓成熟度检测数据集VOC+YOLO格式412张3类别 [数据集][目标检测]雾天行人车辆检测数据集VOC+YOLO格式4415张5类别 [数据集][目标检测]智能手机屏幕缺陷检测数据集VOC+YOLO格式1200张3类别油斑划痕两点三类 [数据集][目标检测]牛羊检测数据集VOC+YOLO格式3393张2类别 [数据集][目标检测]昆虫检测数据集VOC+YOLO格式1873张7类别 [数据集][图像分类]零售行业商品分类数据集256016张357类别 [数据集][目标检测]零售柜零食检测数据集VOC+YOLO格式5422张113类 [数据集][目标检测]螺丝螺母检测数据集VOC+YOLO格式2100张13类别 [数据集][目标检测]城市道路垃圾检测数据集5266张33类别重制版 [数据集][目标检测]遥感图像游泳池检测数据集VOC+YOLO格式288张1类别 [数据集][目标检测]光伏板太阳能板缺陷检测数据集VOC+YOLO格式2400张3类别 [数据集][目标检测]垃圾检测数据集VOC+YOLO格式6004张18类别垃圾 [数据集][目标检测]野生动物检测数据集VOC+YOLO格式1054张4类别 [数据集][图像分类]车部件分类数据集8989张50类别 [数据集][目标检测]小麦病害检测数据集VOC+YOLO格式899张12类别 [数据集][实例分割]小麦病害分割数据集899张12类别 [数据集][图像分类]小麦病害分类数据集7653张12类别 [数据集][图像分类]辣椒病害分类数据集9076张12类别 [数据集][图像分类]棉花叶子病害分类数据集2293张4类别 [数据集][图像分类]大白菜幼叶病害分类数据集2878张3类别 [数据集][图像分类]昆虫分类数据集75222张102类别 [数据集][图像分类]黄瓜叶子病害分类数据集172张3类别 [数据集][图像分类]头发疾病分类数据集12000张10类别 [数据集][图像分类]香蕉叶子病害分类数据集937张4类别 [数据集][图像分类]橙子病害分类数据集1614张4类 [数据集][图像分类]樱桃叶子病害分类数据集4386张2类别 [数据集][图像分类]桃子叶子病害分类数据集4457张2类别 [数据集][图像分类]胡椒叶子病害分类数据集4876张2类别 [数据集][图像分类]玉米叶子病害分类数据集9145张4类别 [数据集][图像分类]葡萄叶子病害分类数据集9027张4类别 [数据集][图像分类]土豆马铃薯叶子病害分类数据集7128张3类别 [数据集][图像分类]草莓叶子病害分类数据集4498张2类别 [数据集][图像分类]西红柿叶子病害分类数据集22930张10类别 [数据集][图像分类]土豆病害分类数据集451张7类别 [数据集][图像分类]苹果叶子病害分类数据集9714张4类别 [数据集][目标检测]芒果叶病害数据集VOC+YOLO格式4000张5类别 [数据集][图像分类]芒果叶病害分类数据集4000张5类别 [数据集][目标检测]红外行人检测数据集VOC+YOLO格式5838张1类别 [数据集][目标检测]无人机高空红外数据集VOC+YOLO格式2866张5类别 [数据集][目标检测]鸡蛋破蛋数据集VOC+YOLO格式792张2类别 [数据集][目标检测]葡萄串检测数据集VOC+YOLO格式95张1类别 [数据集][图像分类]鲜花分类数据集5735张102类别 [数据集][图像分类]中草药分类数据集9983张100类别 [数据集][图像分类]蝴蝶分类数据集13094张100类别 [数据集][目标检测]鸟类检测数据集VOC+YOLO格式11758张200类别 [数据集][目标检测]狗狗表情识别VOC+YOLO格式3971张4类别 [数据集][目标检测]120种犬类狗种类检测数据集VOC+YOLO格式20578张120类别 [数据集][目标检测]课堂举手阅读书写数据集VOC格式5015张3类别 [数据集][目标检测]课堂行为数据集VOC+YOLO格式671张6类别 [数据集][目标检测]游泳者溺水数据集VOC+YOLO格式2类别895张 [数据集][图像分类]垃圾图片分类数据集史上最全233类别共计3.7w张图片 [labelme]labelme如何将标注的json格式转成png的mask文件掩码文件 [数据集][目标检测]茶叶病害数据集VOC+YOLO格式883张8类别 [数据集][目标检测]玩手机检测数据集VOC+YOLO格式8200张1类别 [数据集][目标检测]白细胞检测数据集VOC+YOLO格式343张1类别 [数据集][目标检测]目标检测数据集手势识别VOC+YOLO格式1745张10类别 [数据集][目标检测]车辆检测数据集VOC+YOLO格式1.6w张3类别 [数据集][目标检测]草莓病害数据集VOC+YOLO格式1450张7类别 [数据集][目标检测]昆虫数据集VOC+YOLO格式1938张7类别 [数据集][目标检测]道路缺陷数据集VOC+YOLO格式5000张8类别 [数据集][目标检测]道路缺陷数据集VOC+YOLO格式12195张4类别 [数据集][目标检测]水稻病害目标检测数据集VOC+YOLO格式3355张4类别 [数据集][目标检测]人员持刀数据集VOC+YOLO格式6923张1类别重制版 [数据集][目标检测]电风扇目标检测数据集VOC+YOLO格式364张1类别 [数据集][目标检测]芝麻杂草目标检测数据集VOC+YOLO格式1300张2类别 [数据集][目标检测]道路坑洞目标检测数据集VOC+YOLO格式665张1类别 [数据集][目标检测]小型无人机目标检测数据集VOC+YOLO格式3338张1类别 QQImages头像数据集介绍 [数据集][目标检测]高质量夜间车辆目标检测数据集VOC+YOLO格式6223张3类别 [数据集][目标检测]拉横幅识别横幅检测数据集VOC+yolo格式1962张1类别 [数据集][目标检测]动物目标检测数据集VOC格式+yolo格式超80种类动物 [数据集][目标检测]斑马数据集VOC+yolo格式375张1类别 [数据集][目标检测]犀牛数据集VOC+yolo格式376张1类别 [数据集][目标检测]大象数据集VOC+yolo格式376张1类别 [数据集][目标检测]水牛数据集VOC+yolo格式376张1类别 [数据集][目标检测]街道乱堆物料数据集VOC格式41张1类别 [数据集][目标检测]隔离栅损坏防护栏损坏数据集VOC-28张1类别 [数据集][目标检测]智慧城市沿街晾晒检测数据集VOC+YOLO格式625张1类别 [数据集][目标检测]垃圾桶溢出数据集VOC+YOLO格式3类别3493张 [目标检测][数据集]电瓶车进电梯电动车入梯电动车进电梯数据集727张3类别 [数据集][目标检测]消防栓目标检测数据集VOC+YOLO格式2600张1类别 [数据集][目标检测]裸土识别裸土未覆盖目标检测数据集VOC+YOLO格式857张2类别 [数据集][目标检测]疲劳驾驶数据集VOC格式4类别-4362张 [数据集][目标检测]垃圾目标检测数据集VOC格式14963张44类别 [数据集][目标检测]骑电动车摩托车不戴头盔数据集VOC格式1385张 [数据集][目标检测]PCB板缺陷目标检测数据集VOC格式693张6类别 [数据集][目标检测]道路坑洼目标检测数据集VOC格式1510张2类别 [数据集][目标检测]钢材表面缺陷目标检测数据集VOC格式2279张10类别 [数据集][目标检测]条码目标检测数据集VOC格式15442张 [数据集][目标检测]二维码目标检测数据集VOC格式2589张 [数据集][目标检测]遛狗不牵绳数据集VOC格式-1980张 [数据集][目标检测]城市道路井盖破损丢失数据集VOC+YOLO格式1377张4类别 [数据集][目标检测]危化品运输车数据集油罐车危化品运输车辆目标检测数据集2007张 [数据集][目标检测]开车未系安全带目标检测数据集voc-190张 [数据集][目标检测]天牛数据集目标检测数据集VOC格式3050张 [数据集][目标检测]公路落石和滑坡数据集VOC格式-991张 [数据集][目标检测]目标检测数据集蜜蜂4073张1类别VOC格式 [数据集][目标检测]目标检测数据集绝缘子缺陷防震锤1688张5类别VOC格式 [数据集][目标检测]数据集VOC格式绝缘子缺陷检测数据集VOC-4086张 [数据集][目标检测]目标检测数据集大白菜数据集VOC格式1557张 [数据集][目标检测]目标检测数据集黄瓜数据集VOC格式1309张 [数据集][目标检测]篮球数据集VOC格式7398张 [数据集][目标检测]塔吊数据集VOC格式2559张 [数据集介绍][目标检测]城市道路交通事故数据集VOC格式1182张 [数据集介绍][目标检测]城市街道垃圾数据集VOC格式5266张 [数据集][VOC][目标检测]河道垃圾水面漂浮物数据集目标检测可用yolo训练-1304张介绍 [数据集][VOC][目标检测]输电线异物数据集目标检测可用yolo训练-4165张介绍 [数据集][VOC][目标检测]翻越栏杆翻越防护栏数据集目标检测可用yolo训练-1035张介绍 [数据集][VOC][目标检测]西瓜数据集目标检测可用yolo训练-1702张介绍 [数据集][VOC][目标检测]人脸表情识别目标检测数据集3.2w张介绍 [深度学习][数据集][目标检测]工程车辆数据集16881张15种类别车辆介绍 [数据集][VOC]挖掘机数据集voc格式4288张介绍 [数据集][VOC]高质量的目标检测数据集合集(持续更新) [数据集][VOC]消防灭火器数据集voc格式5156张介绍 [数据集][VOC]张贴小广告数据集voc格式1725张 [数据集][VOC]老鼠数据集voc格式3001张 [数据集][VOC]男女数据集voc格式6188张 常见30种鱼类分类数据集1917张30类别 城市道路井盖破损丢失目标检测数据集VOC+YOLO格式1377张4类别 [数据集][目标检测]谢韦尔钢材缺陷检测数据集VOC+YOLO格式6666张4类别 铁路列车手势分类数据集3852张5类别 航拍水体污染检测数据集VOC+YOLO格式2999张5类别 [数据集][目标检测]水稻叶片病害检测数据集VOC+YOLO格式1448张3类别 蛋壳裂缝检测数据集VOC+YOLO格式2458张2类别 NEU-DET钢材表面缺陷检测数据集VOC+YOLO格式1799张6类别 鲜花雏菊蒲公英玫瑰向日葵郁金香分类数据集4326张5类别 服装布匹缺陷检测数据集VOC+YOLO格式8073张4类别 昆虫检测数据集VOC+YOLO格式9348张102类别 煤矸石检测数据集VOC+YOLO格式3090张2类别 煤矿输送带异物检测数据集VOC+YOLO格式2220张2类别 玉米地杂草检测数据集VOC+YOLO格式7436张2类别 路面垃圾检测数据集VOC+YOLO格式8097张27类别 打架行为检测数据集VOC+YOLO格式7327张2类别 昆虫检测数据集VOC+YOLO格式9348张102类别 脑瘤检测数据集VOC+YOLO格式9973张4类别 [数据集][图像分类]蔬菜分类数据集2.1万张15类豆子苦瓜葫芦茄子西兰花卷心菜辣椒胡萝卜花椰菜黄瓜木瓜土豆南瓜萝卜和西红柿 电力场景光伏板航拍鸟粪缺陷检测数据集VOC+YOLO格式367张1类别 骨折检测数据集VOC+YOLO格式2060张7类 电力场景输电线路红外过热检测数据集VOC+YOLO格式2253张1类别 电力场景配电变压器检测数据集VOC+YOLO格式2994张1类别 电力场景输电线路螺栓销钉缺失检测数据集VOC+YOLO格式2504张4类别 电力场景变电站控制柜状态检测数据集VOC+YOLO格式3000张16类别 [数据集][目标检测]电力场景红外图像开关柜接头过热部件检测数据集VOC+YOLO格式4271张14类别 [数据集][目标检测]电力场景配电柜光按钮检测数据集VOC+YOLO格式769张1类别 电力场景变电站继电保护控制柜屏幕检测数据集VOC+YOLO格式1103张6类别 电力场景红外图像电力设备部件检测数据集VOC+YOLO格式3930张7类别 电力场景变电站红外图像电压电流互感器检测数据集VOC+YOLO格式889张7类别 红火蚁检测数据集VOC+YOLO格式9505张1类别 铝型材表面缺陷检测数据集VOC+YOLO格式1793张10类别 [数据集][目标检测]航拍路面病害检测道路裂缝检测数据集VOC+YOLO格式3302张7类别 [数据集][目标检测]智慧牧场航拍牛羊检测远距离小目标数据集VOC+YOLO格式1021张3类别 [数据集][目标检测]鸟类检测数据集VOC+YOLO格式16283张10类别 电力场景各种部件检测数据集VOC+YOLO格式10559张18类别 猪场监控猪只吃睡检测数据集VOC+YOLO格式9652张3类别 绝缘子缺陷检测数据集VOC+YOLO格式2139张9类别 电表读数检测数据集VOC+YOLO格式1199张12类别 [数据集][目标检测]电表读数检测数据集VOC+YOLO格式3573张12类别 [数据集][目标检测]齿轮检测数据集VOC+YOLO格式544张6类别 [数据集][目标检测]齿轮缺陷检测数据集VOC+YOLO格式2978张3类别 WiderPerson密集行人检测数据集VOC+YOLO格式9000张1类别送yolov5模型 打架识别分类数据集75855张8类别 火星月球陨石坑检测数据集VCO+YOLO格式132张1类别 [数据集][图像分类]濒危动物野生动物分类数据集5400张90类别 bdd100k数据集VOC+YOLO格式79850张13类别 皮革缺陷分类数据集3600张6类别 疟疾细胞感染分类数据集27560张2类别 双单核细胞检测数据集VOC+YOLO格式2609张6类别 血细胞类型分类数据集12507张4类别 交通标志检测CCTSDB数据集VOC+YOLO格式8719张3类别 野生猫科动物分类数据集2389张10类别 62种野外可食用植物分类数据集4665张62类别 风力机缺陷检测损伤检测数据集VOC+YOLO格式3687张1类别 智能垃圾分拣车垃圾检测数据集VOC+YOLO格式500张5类别 输电线路涉鸟故障相关鸟种细粒度分类数据集2889张50类别 配电室设备对象检测数据集VOC+YOLO格式153张34类别 101类美食分类数据集101000张101类别 番茄西红柿叶子病害分类数据集12882张11类别 钢筋长度超限检测检数据集VOC+YOLO格式215张1类别 桃脸识别桃子分拣分类数据集6704张4类别 共享单车检测数据集VOC+YOLO格式136张1类别 斑马线人行横道检测数据集VOC+YOLO格式192张1类别 轮胎缺陷分类数据集495张2类别 动力电池隔膜缺陷分类数据集35698张5类别 苹果缺陷检测数据集VOC+YOLO格式8978张2类别 摄像头缺陷检测数据集VOC+YOLO格式988张4类别 药丸的缺陷检测数据集VOC+YOLO格式2759张3类别 钢材表面缺陷检测数据集VOC+YOLO格式2280张10类别 喷粉缺陷检测数据集VOC+YOLO格式12926张1类别 轮胎X光缺陷检测数据集VOC+YOLO格式1903张4类别 香烟缺陷检测数据集VOC+YOLO格式197张2类别 纸板缺陷检测数据集VOC+YOLO格式1055张1类别 半导体晶圆缺陷图谱分类数据集25519张8类别 小麦穗检测数据集VOC+YOLO格式1691张1类别 水下垃圾检测数据集VOC+YOLO格式7666张13类别 手势分类数据集5790张10类别.zip 绵羊脸部绵羊品种分类数据集1680张4类别 猪脸分类识别数据集5909张30类别 猪脸检测数据集VOC+YOLO格式217张2类别 新冠肺炎CT胸透识别分类数据集4035张3类别 光伏电池异常检测数据集VOC+YOLO格式219张2类别 皮肤癌病变分类数据集2357张9类别 皮肤病识别分类数据集23020张26类别 邮件盒快递盒子检测数据集VOC+YOLO格式1037张1类别 快递包裹纸箱检测数据集VOC+YOLO格式395张2类别 医学OCT视网膜疾病识别分类数据集14600张4类别 flavia植物叶片分类数据集1907张32类别 香蕉新鲜度等级识别香蕉成熟度识别分类数据集1186张3类别 农作物害虫识别分类数据集4183张7类别 眼疾分类数据集1800张6类别 大豆叶子病害分类数据集1296张3类别 道路路标交通标志检测数据集VOC+YOLO格式877张4类别 钢材缺陷检测数据集VOC+YOLO格式386张5类别 【目标检测数据集】摩托车数据集3500张VOC+YOLO格式 【目标检测数据集】安全帽检测VOC+YOLO格式1600张 目标检测数据集】烟雾明火目标检测数据集3000张VOC+YOLO格式 【目标检测数据集】电力行业高空作业安全带检测数据集VOC+YOLO格式147张4类别 【目标检测数据集】鸟数据集3233张VOC+YOLO格式 【目标检测数据集】草莓成熟度度检测数据集VOC+YOLO格式400张 【目标检测数据集】布匹缺陷检测数据集VOC+YOLO格式 【目标检测数据集】自行车数据集3250张VOC+YOLO格式 目标检测数据集】牛检测数据集1968张VOC+YOLO格式 【目标检测数据集】草莓成熟度度检测数据集VOC+YOLO格式400张 【目标检测数据集】布匹缺陷检测数据集VOC+YOLO格式 【目标检测数据集】自行车数据集3250张VOC+YOLO格式 【目标检测数据集】盆栽植物4452张VOC+YOLO格式 【目标检测数据集】椅子数据集12700张VOC+YOLO格式 【目标检测数据集】遥控器数据集3076张VOC+YOLO格式 【目标检测数据集】羊检测数据集1529张VOC+YOLO格式 【目标检测数据集】披萨数据集3166张VOC+YOLO格式 牙刷数据集1000张VOC+YOLO格式 【目标检测数据集】熊数据集960张VOC+YOLO格式 【目标检测数据集】消防栓检测数据集1711张VOC+yolo格式 【目标检测数据集】苹果数据集1586张VOC+YOLO格式 【目标检测数据集】香蕉数据集2240张VOC+YOLO格式 【目标检测数据集】西蓝花数据集1930张VOC+YOLO格式 【目标检测数据集】微波炉数据集1547张VOC+YOLO格式 咖啡豆缺陷分类数据集1313张3类别 运动分类数据集14572张100类别 运动分类数据集11146张73类别 csgo检测人和头数据集10024张2类别 【目标检测数据集】瓶子数据集4000张VOC+YOLO格式 【目标检测数据集】网球拍数据集3350张VOC+YOLO格式 甜甜圈数据集1523张VOC+YOLO格式 【目标检测数据集】泰迪熊数据集2140张VOC+YOLO格式 【目标检测数据集】热狗数据集1200张VOC+YOLO格式 【目标检测数据集】鼠标数据集1876张VOC+YOLO格式 【目标检测数据集】书籍数据集5000张VOC+YOLO格式 【目标检测数据集】手提包数据集6840张VOC+YOLO格式 【目标检测数据集】三明治数据集2365张VOC+YOLO格式 沙发数据集4423张VOC+YOLO格式 【目标检测数据集】时钟数据集4600张VOC+YOLO格式 【目标检测数据集】手机检测数据集4801张VOC+YOLO格式 中国交通标志检测数据集VOC+YOLO格式5998张58类别 矿石分类数据集929张7类别 车辆分类数据集1600张10类别 道路交通事故检测数据集VOC+YOLO格式16641张2类别 道路交通事故检测数据集VOC+YOLO格式341张3类别 钢丝绳破损灼伤缺陷检测数据集VOC+YOLO格式1318张2类别 Open Images v7数据集介绍 输电线异物检测数据集VOC+YOLO格式1300张4类别 传送带异物检测及物料跑偏检测数据集VOC+YOLO格式437张3类别 工业仪表盘检测数据集VOC+YOLO格式783张1类别 脑肿瘤分类数据集7023张4类别 杂草分类数据集5998张5类别 水稻慈姑类杂草检测数据集221张2类别 小辣椒小彩椒检测数据集2292张3类别 电力行业高空作业安全带检测数据集VOC+YOLO格式147张4类别 高空作业及安全带佩戴检测数据集VOC+YOLO格式2546张4类别 石头分类数据集2087张7类 舌头舌像检测数据集VOC+YOLO格式800张5类别 布匹缺陷检测数据集VOC+YOLO格式4774张34类别 剪刀石头布分类数据集2188张3类别 [数据集][目标检测]条码目标检测数据集VOC格式15442张 水稻病害分类数据集1886张8类别 木薯叶病害分类数据集21397张5类别 昆虫检测数据集VOC+YOLO格式1873张7类别
魔搭社区 收录
中国区域地面气象要素驱动数据集 v2.0(1951-2020)
中国区域地面气象要素驱动数据集(China Meteorological Forcing Data,以下简称 CMFD)是为支撑中国区域陆面、水文、生态等领域研究而研发的一套高精度、高分辨率、长时间序列数据产品。本页面发布的 CMFD 2.0 包含了近地面气温、气压、比湿、全风速、向下短波辐射通量、向下长波辐射通量、降水率等气象要素,时间分辨率为 3 小时,水平空间分辨率为 0.1°,时间长度为 70 年(1951~2020 年),覆盖了 70°E~140°E,15°N~55°N 空间范围内的陆地区域。CMFD 2.0 融合了欧洲中期天气预报中心 ERA5 再分析数据与气象台站观测数据,并在辐射、降水数据产品中集成了采用人工智能技术制作的 ISCCP-ITP-CNN 和 TPHiPr 数据产品,其数据精度较 CMFD 的上一代产品有显著提升。 CMFD 历经十余年的发展,其间发布了多个重要版本。2019 年发布的 CMFD 1.6 是完全采用传统数据融合技术制作的最后一个 CMFD 版本,而本次发布的 CMFD 2.0 则是 CMFD 转向人工智能技术制作的首个版本。此版本与 1.6 版具有相同的时空分辨率和基础变量集,但在其它诸多方面存在大幅改进。除集成了采用人工智能技术制作的辐射和降水数据外,在制作 CMFD 2.0 的过程中,研发团队尽可能采用单一来源的再分析数据作为输入并引入气象台站迁址信息,显著缓解了 CMFD 1.6 中因多源数据拼接和气象台站迁址而产生的虚假气候突变。同时,CMFD 2.0 数据的时间长度从 CMFD 1.6 的 40 年大幅扩展到了 70 年,并将继续向后延伸。CMFD 2.0 的网格空间范围虽然与 CMFD 1.6 相同,但其有效数据扩展到了中国之外,能够更好地支持跨境区域研究。为方便用户使用,CMFD 2.0 还在基础变量集之外提供了若干衍生变量,包括近地面相对湿度、雨雪分离降水产品等。此外,CMFD 2.0 摒弃了 CMFD 1.6 中通过 scale_factor 和 add_offset 参数将实型数据化为整型数据的压缩技术,转而直接将实型数据压缩存储于 NetCDF4 格式文件中,从而消除了用户使用数据时进行解压换算的困扰。 本数据集原定版本号为 1.7,但鉴于本数据集从输入数据到研制技术都较上一代数据产品有了大幅的改变,故将其版本号重新定义为 2.0。CMFD 2.0 的数据内容与此前宣传的 CMFD 1.7 基本一致,仅对 1983 年 7 月以后的向下短/长波辐射通量数据进行了更新,以修正其长期趋势存在的问题。2021 年至 2024 年的 CMFD 数据正在制作中,计划于 2025 年上半年发布,从而使 CMFD 2.0 延伸至 2024 年底。
国家青藏高原科学数据中心 收录