WeatherBench 2|天气预报数据集|数据驱动模型数据集
收藏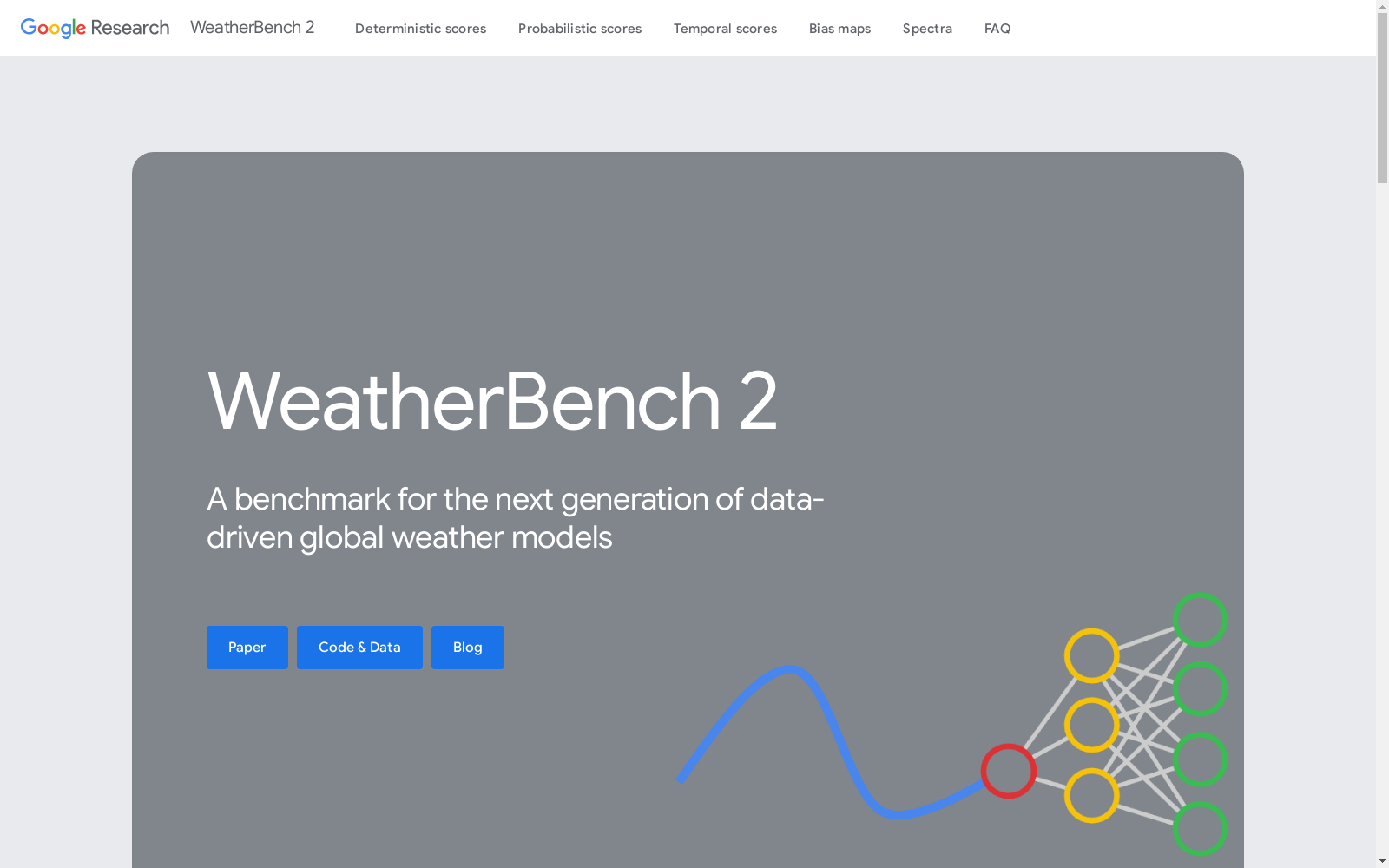
UIEB, U45, LSUI
本仓库提供了水下图像增强方法和数据集的实现,包括UIEB、U45和LSUI等数据集,用于支持水下图像增强的研究和开发。
github 收录
MedDialog
MedDialog数据集(中文)包含了医生和患者之间的对话(中文)。它有110万个对话和400万个话语。数据还在不断增长,会有更多的对话加入。原始对话来自好大夫网。
github 收录
Beijing Traffic
The Beijing Traffic Dataset collects traffic speeds at 5-minute granularity for 3126 roadway segments in Beijing between 2022/05/12 and 2022/07/25.
Papers with Code 收录
Breast Cancer Dataset
该项目专注于清理和转换一个乳腺癌数据集,该数据集最初由卢布尔雅那大学医学中心肿瘤研究所获得。目标是通过应用各种数据转换技术(如分类、编码和二值化)来创建一个可以由数据科学团队用于未来分析的精炼数据集。
github 收录
CMNEE(Chinese Military News Event Extraction dataset)
CMNEE(Chinese Military News Event Extraction dataset)是国防科技大学、东南大学和清华大学联合构建的一个大规模的、基于文档标注的开源中文军事新闻事件抽取数据集。该数据集包含17,000份文档和29,223个事件,所有事件均基于预定义的军事领域模式人工标注,包括8种事件类型和11种论元角色。数据集构建遵循两阶段多轮次标注策略,首先通过权威网站获取军事新闻文本并预处理,然后依据触发词字典进行预标注,经领域专家审核后形成事件模式。随后,通过人工分批、迭代标注并持续修正,直至满足既定质量标准。CMNEE作为首个专注于军事领域文档级事件抽取的数据集,对推动相关研究具有显著意义。
github 收录