kaist-ai/Multifaceted-Collection-ORPO|语言模型训练数据集|偏好对齐数据集
收藏数据集概述
数据集详情
数据集结构
数据字段
main_source
(字符串): 指令的源数据集original_source
(字符串): 根据源数据集的指令原始来源system
(字符串): 系统消息,详细说明遵循个人多方面偏好的目标,涉及四个高层次的理想响应维度(风格、背景知识、信息量和无害性)prompt
(字符串): 指示特定任务的指令chosen
(列表[字典[字符串, 字符串]]): 最佳遵循系统消息和指令的黄金响应,由gpt-4-0125-preview
生成。格式化为对话,即[{"content": ..., "role": "user"}, {"content": ..., "role": "assistant"}]
rejected
(列表[字典[字符串, 字符串]]): 最佳遵循不同系统消息和指令的黄金响应,由gpt-4-0125-preview
生成。格式与chosen
相同。
数据实例
以下是数据集中的一个示例实例:
json { "main_source": "OpenHermesPreferences", "original_source": "OpenHermesPreferences", "system": "You are a persuasive environmental advocate, tasked with enlightening individuals on the intricacies of climate change, making a compelling case for urgent collective action. Equipped with the assumption that your audience possesses a foundational, yet incomplete, understanding of climate change, you bridge knowledge gaps without burdening them with excessive complexity. Your narrative weaves together a concise yet comprehensive overview of climate change, including its causes, consequences, and humanitys role within this global issue, ensuring a balance between depth and brevity. With a keen sensitivity to environmental justice, you underscore how the ramifications of climate change disproportionately affect the most vulnerable communities, advocating for an inclusive approach that recognizes the interplay between environmental challenges and societal inequities. Your goal is to not only inform but also mobilize, harnessing the power of persuasive communication to foster a sense of responsibility and immediate action towards mitigating the effects of climate change.", "prompt": "Edit the given paragraph for grammar and style, making it more engaging and concise. Climate changes rapidly in response to alterations in Earths atmosphere. The activities of humans plays a large role in atmosphere changes, and thus leads to global warming. More particularly, the release in huge amounts of CO2 by burning fossil fuels, industrial processes, and deforestation are some of the main factors. The adverse consequences of global warming are numerous, affecting the environment and human life, including devastating weather events, ocean levels rising, and negatively impacting the habitat of many species.", "chosen": [ { "content": "Edit the given paragraph for grammar and style, making it more engaging and concise. Climate changes rapidly in response to alterations in Earths atmosphere. The activities of humans plays a large role in atmosphere changes, and thus leads to global warming. More particularly, the release in huge amounts of CO2 by burning fossil fuels, industrial processes, and deforestation are some of the main factors. The adverse consequences of global warming are numerous, affecting the environment and human life, including devastating weather events, ocean levels rising, and negatively impacting the habitat of many species.", "role": "user" }, { "content": "Climate change accelerates with shifts in Earths atmosphere, significantly driven by human activities. The burning of fossil fuels, industrial operations, and deforestation unleash vast amounts of CO2, propelling global warming. This warming leads to dire consequences for both the environment and humanity: devastating weather phenomena, rising ocean levels, and the jeopardization of numerous species habitats.", "role": "assistant" } ], "rejected": [ { "content": "Edit the given paragraph for grammar and style, making it more engaging and concise. Climate changes rapidly in response to alterations in Earths atmosphere. The activities of humans plays a large role in atmosphere changes, and thus leads to global warming. More particularly, the release in huge amounts of CO2 by burning fossil fuels, industrial processes, and deforestation are some of the main factors. The adverse consequences of global warming are numerous, affecting the environment and human life, including devastating weather events, ocean levels rising, and negatively impacting the habitat of many species.", "role": "user" }, { "content": "Climate swiftly shifts in response to changes in Earths atmosphere, with human activities at the heart of these transformations, fueling global warming. Notably, the massive release of CO2 through burning fossil fuels, industrial endeavors, and deforestation stands as a principal culprit. The fallout of global warming is vast, imperiling both the environment and human well-being. It manifests in catastrophic weather events, rising ocean levels, and the disruption of habitats for countless species.", "role": "assistant" } ] }
数据集创建
数据收集和处理
-
指令采样:
- 从五个高质量偏好数据集中选择指令:
- 移除完全重复项,并使用正则表达式模式过滤具有预先存在系统消息的指令,最终得到65k条指令。
-
偏好集生成:
- 初始识别四个主要响应偏好维度:风格、背景知识、信息量和无害性。
- 定义一个包含每个维度一个偏好的偏好集。
- 对于每个65k条指令,使用
gpt-4-0125-preview
生成3个不同的任务对齐偏好集。
-
系统消息和黄金响应生成:
- 使用GPT-4 Turbo将每个偏好集转换为系统消息,每个指令生成三个系统消息。
- 再次使用GPT-4 Turbo为每个系统消息制作黄金标准的多方面响应。
数据集摘要
Multifaceted Collection ORPO是一个适用于几率比偏好优化(ORPO)的训练数据集。该数据集包含65k条独特的指令,每个指令选择一个系统消息,并将对齐的响应标记为“chosen”,同时从其余两个非对齐系统消息中选择一个响应标记为“rejected”。
- 许可证:Creative Commons Attribution 4.0
- 相关数据集:Multifaceted-Collection, Multifaceted-Collection-DPO, Multifaceted-Collection-RM, Multifaceted-Bench
- 训练模型:Janus-ORPO-7B
数据集信息
- 特征:
main_source
(字符串)original_source
(字符串)system
(字符串)prompt
(字符串)chosen
(列表)rejected
(列表)
- 分割:
train
:507621991字节,64641个样本
- 下载大小:285644717字节
- 数据集大小:507621991字节
- 配置:
default
:数据文件路径为data/train-*
- 许可证:cc-by-4.0
- 任务类别:text-generation
- 大小类别:10K<n<100K
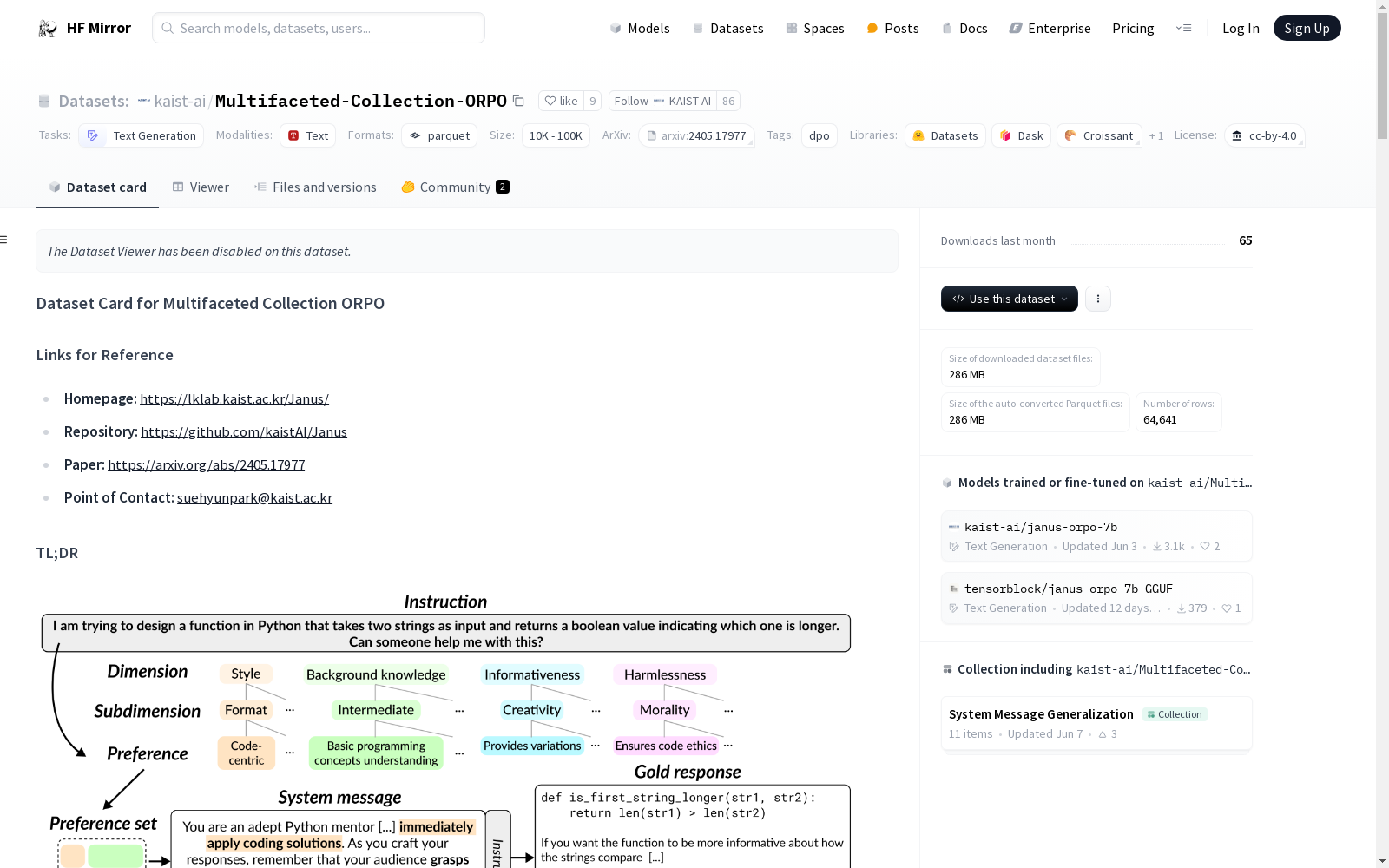
中国气象数据
本数据集包含了中国2023年1月至11月的气象数据,包括日照时间、降雨量、温度、风速等关键数据。通过这些数据,可以深入了解气象现象对不同地区的影响,并通过可视化工具揭示中国的气温分布、降水情况、风速趋势等。
github 收录
Yahoo Finance
Dataset About finance related to stock market
kaggle 收录
AISHELL/AISHELL-1
Aishell是一个开源的中文普通话语音语料库,由北京壳壳科技有限公司发布。数据集包含了来自中国不同口音地区的400人的录音,录音在安静的室内环境中使用高保真麦克风进行,并下采样至16kHz。通过专业的语音标注和严格的质量检查,手动转录的准确率超过95%。该数据集免费供学术使用,旨在为语音识别领域的新研究人员提供适量的数据。
hugging_face 收录
LIDC-IDRI
LIDC-IDRI 数据集包含来自四位经验丰富的胸部放射科医师的病变注释。 LIDC-IDRI 包含来自 1010 名肺部患者的 1018 份低剂量肺部 CT。
OpenDataLab 收录
ISIC 2018
ISIC 2018数据集包含2594张皮肤病变图像,用于皮肤癌检测任务。数据集分为训练集、验证集和测试集,每张图像都附有详细的元数据,包括病变类型、患者年龄、性别和解剖部位等信息。
challenge2018.isic-archive.com 收录