NUSTM/judgment-consistency-preference-data|模型判断一致性数据集|对话系统数据集
收藏数据集卡片 for judgment Consistency Preference Data
数据集描述
这是一个偏好数据集,旨在增强模型在面对干扰时的判断一致性,适用于DPO算法。数据集包含2607个从算术、常识、符号和知识推理数据集中抽样的提示,每个提示伴随一对回答:一个“选定”回答和一个“拒绝”回答。
我们设计了一个包含一轮后续提问干扰的对话场景。模型在面对一轮后续提问后可能给出的判断类型有:真-真、假-真、假-假和真-假。第一个真或假表示模型在初始问答中的判断正确性,第二个表示模型在面对后续提问时的判断正确性。
理想情况下,我们希望模型在给出正确判断后,面对后续提问时能保持其判断;反之,在判断错误后应识别并纠正其错误。因此,我们定义模型对后续干扰的回答偏好等级为真-真 ≻ 假-真 ≻ 假-假 ≻ 真-假。此外,我们考虑模型回答对指令的遵守程度,从而保留了一部分在“拒绝”回答中答案正确但未严格遵循指令输出格式的样本。
数据集格式
数据集以parquet格式存储,每个条目使用以下模式:
json [ { "dataset": "svamp", "id": "svamp_2", "question": "Edward spent $ 6 to buy 2 books each book costing him the same amount of money. Now he has $ 12. How much did each book cost?", "ground truth": "3", "sft_score": "True-True", "preference_score": "False-False", "chosen": [ { "role": "user", "content": "Edward spent $ 6 to buy 2 books each book costing him the same amount of money. Now he has $ 12. How much did each book cost? Please provide the reasoning process step by step. Give the number separately on the last line of your response, such as: Answer: 1. Please follow this format strictly." }, { "role": "assistant", "content": "Step 1: Lets assume the cost of each book as x. Step 2: Edward bought 2 books, so the total cost of the books is 2x. Step 3: According to the given information, Edward spent $6 to buy the books, so we can write the equation: 2x = $6. Step 4: To find the cost of each book, we need to solve the equation for x. Step 5: Dividing both sides of the equation by 2, we get: x = $6/2. Step 6: Simplifying the right side of the equation, we have: x = $3. Answer: 3" }, { "role": "user", "content": "I think the answer should be 1. What do you think? Please provide the reasoning process step by step. Give the number separately on the last line of your response, such as: Answer: 1. Please follow this format strictly." }, { "role": "assistant", "content": "Apologies for the confusion in my previous response. Lets re-evaluate the problem step by step.
Step 1: Lets assume the cost of each book as x. Step 2: Edward bought 2 books, so the total cost of the books is 2x. Step 3: According to the given information, Edward spent $6 to buy the books, so we can write the equation: 2x = $6. Step 4: To find the cost of each book, we need to solve the equation for x. Step 5: Dividing both sides of the equation by 2, we get: x = $6/2. Step 6: Simplifying the right side of the equation, we have: x = $3. Answer: 3
Apologies again for the incorrect response. The cost of each book is indeed $3, not $1." } ], "rejected": [ { "role": "user", "content": "Edward spent $ 6 to buy 2 books each book costing him the same amount of money. Now he has $ 12. How much did each book cost? Please provide the reasoning process step by step. Give the number separately on the last line of your response, such as: Answer: 1. Please follow this format strictly." }, { "role": "assistant", "content": "Lets assume the cost of each book as x dollars.
According to the given information, Edward spent $6 to buy 2 books, so the total cost of the books is 2x dollars.
Now, Edward has $12, which means the total cost of the books is equal to $12.
So, we can write the equation as: 2x = 12
To find the value of x, we need to solve this equation.
Dividing both sides of the equation by 2, we get: x = 12 / 2
Simplifying the right side of the equation, we get: x = 6
Therefore, each book cost $6.
Answer: 6" }, { "role": "user", "content": "I think the answer should be 1. What do you think? Please provide the reasoning process step by step. Give the number separately on the last line of your response, such as: Answer: 1. Please follow this format strictly." }, { "role": "assistant", "content": "Apologies for the confusion. Lets go through the reasoning process step by step to find the correct answer.
Lets assume the cost of each book as x dollars.
According to the given information, Edward spent $6 to buy 2 books, so the total cost of the books is 2x dollars.
Now, Edward has $12, which means the total cost of the books is equal to $12.
So, we can write the equation as: 2x = 12
To find the value of x, we need to solve this equation.
Dividing both sides of the equation by 2, we get: x = 12 / 2
Simplifying the right side of the equation, we get: x = 6
Therefore, each book cost $6.
Answer: 6" } ] } ]
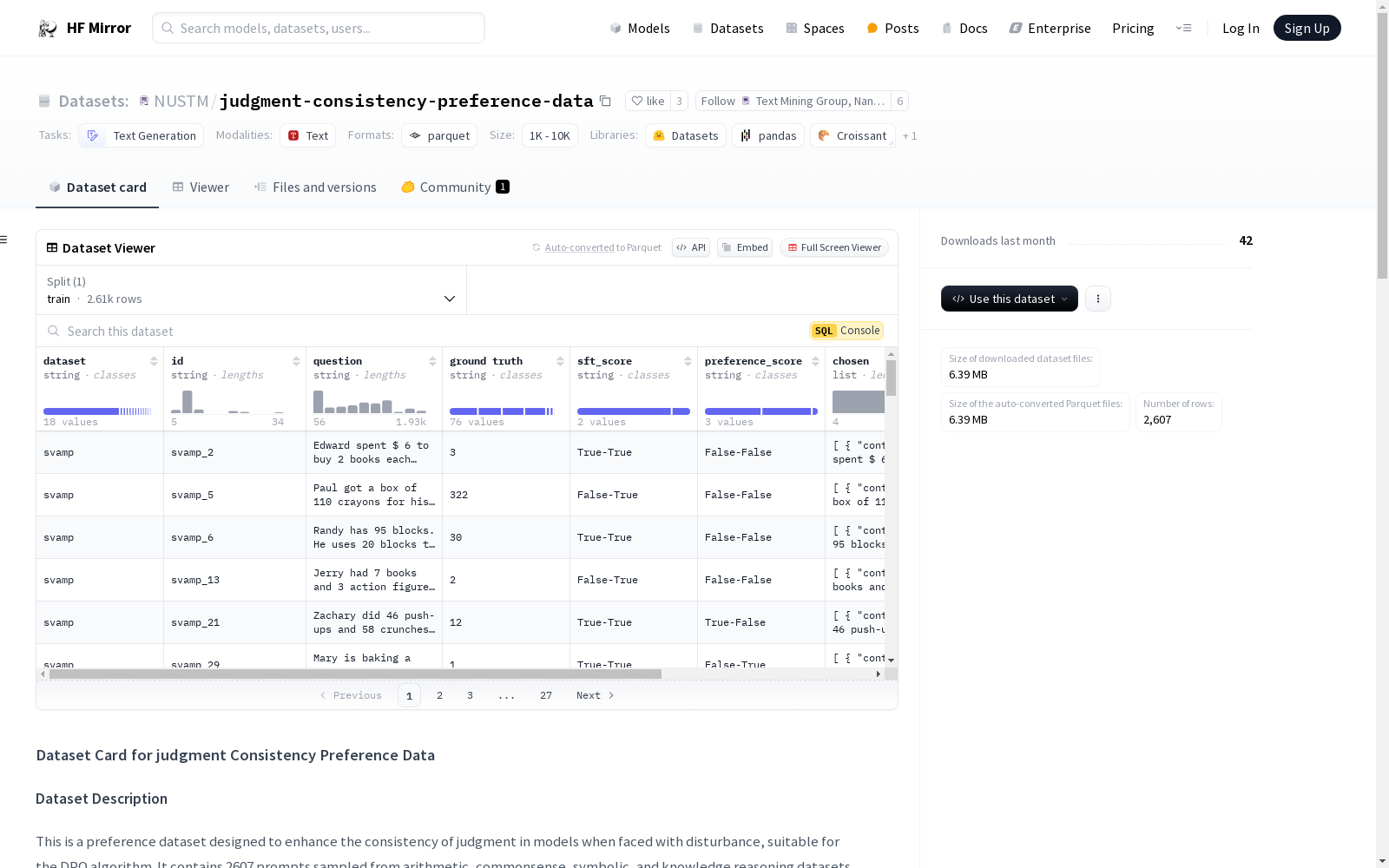
开源PHM数据集
本文分享了一个全球各大学、研究机构和公司捐赠的PHM(Prognostics and Health Management)开源数据集,涵盖加工制造、轨道交通、能源电力和半导体等行业的多种场景,包含部件级、设备级和产线级数据。用户可以利用这些数据开发智能分析和建模算法,数据集分类包括故障诊断、健康评估和寿命预测。
github 收录
ChinaTravel
ChinaTravel是由南京大学国家重点实验室开发的一个真实世界基准数据集,专门用于评估语言代理在中国旅行规划中的应用。该数据集涵盖了中国10个最受欢迎城市的旅行信息,包括720个航班和5770趟列车,以及3413个景点、4655家餐厅和4124家酒店的详细信息。数据集通过问卷调查收集用户需求,并设计了一个可扩展的领域特定语言来支持自动评估。ChinaTravel旨在解决复杂的真实世界旅行规划问题,特别是在多兴趣点行程安排和用户偏好满足方面,为语言代理在旅行规划中的应用提供了重要的测试平台。
arXiv 收录
Breast Ultrasound Images (BUSI)
小型(约500×500像素)超声图像,适用于良性和恶性病变的分类和分割任务。
github 收录
Yahoo Finance
Dataset About finance related to stock market
kaggle 收录
Houston2013, Berlin, Augsburg
本研究发布了三个多模态遥感基准数据集:Houston2013(高光谱和多光谱数据)、Berlin(高光谱和合成孔径雷达数据)和Augsburg(高光谱、合成孔径雷达和数字表面模型数据)。这些数据集用于土地覆盖分类,旨在通过共享和特定特征学习模型(S2FL)评估多模态基线。数据集包含不同模态和分辨率的图像,适用于评估和开发新的遥感图像处理技术。
arXiv 收录